This is the multi-page printable view of this section. Click here to print.
Postgres
- Pig, The Postgres Extension Wizard
- The idea way to install PostgreSQL Extensions
- Self-Hosting Dify with PG, PGVector, and Pigsty
- PGCon.Dev 2024, The conf that shutdown PG for a week
- Postgres is eating the database world
- PostgreSQL Convention 2024
- PostgreSQL, The most successful database
Pig, The Postgres Extension Wizard
Title: Meet Pig: The Postgres Extension Wizard
Ever wished installing or upgrading PostgreSQL extensions didn’t feel like digging through outdated readmes, cryptic configure scripts, or random GitHub forks & patches? The painful truth is that Postgres’s richness of extension often comes at the cost of complicated setups—especially if you’re juggling multiple distros or CPU architectures.
Enter Pig, a Go-based package manager built to tame Postgres and its ecosystem of 400+ extensions in one fell swoop. TimescaleDB, Citus, PGVector, 20+ Rust extensions, plus every must-have piece to self-host Supabase — Pig’s unified CLI makes them all effortlessly accessible. It cuts out messy source builds and half-baked repos, offering version-aligned RPM/DEB packages that work seamlessly across Debian, Ubuntu, and RedHat flavors. No guesswork, no drama.
Instead of reinventing the wheel, Pig piggyback your system’s native package manager (APT, YUM, DNF) and follow official PGDG packaging conventions to ensure a glitch-free fit. That means you don’t have to choose between “the right way” and “the quick way”; Pig respects your existing repos, aligns with standard OS best practices, and fits neatly alongside other packages you already use.
Ready to give your Postgres superpowers without the usual hassle? Check out GitHub for documentation, installation steps, and a peek at its massive extension list. Then, watch your local Postgres instance transform into a powerhouse of specialized modules—no black magic is required. If the future of Postgres is unstoppable extensibility, Pig is the genie that helps you unlock it. Honestly, nobody ever complained that they had too many extensions.
PIG v0.1 Release | GitHub Repo | Blog: The Idea Way to deliver PG Extensions
Get Started
Install the pig
package itself with scripts or the traditional yum/apt way.
curl -fsSL https://repo.pigsty.io/pig | bash
Then it’s ready to use; assume you want to install the pg_duckdb
extension:
$ pig repo add pigsty pgdg -u # add pgdg & pigsty repo, update cache
$ pig repo set -u # overwrite all existing repos, brute but effective
$ pig ext install pg17 # install native PGDG PostgreSQL 17 kernels packages
$ pig ext install pg_duckdb # install the pg_duckdb extension (for current pg17)
Extension Management
pig ext list [query] # list & search extension
pig ext info [ext...] # get information of a specific extension
pig ext status [-v] # show installed extension and pg status
pig ext add [ext...] # install extension for current pg version
pig ext rm [ext...] # remove extension for current pg version
pig ext update [ext...] # update extension to the latest version
pig ext import [ext...] # download extension to local repo
pig ext link [ext...] # link postgres installation to path
pig ext build [ext...] # setup building env for extension
Repo Management
pig repo list # available repo list (info)
pig repo info [repo|module...] # show repo info (info)
pig repo status # show current repo status (info)
pig repo add [repo|module...] # add repo and modules (root)
pig repo rm [repo|module...] # remove repo & modules (root)
pig repo update # update repo pkg cache (root)
pig repo create # create repo on current system (root)
pig repo boot # boot repo from offline package (root)
pig repo cache # cache repo as offline package (root)
The idea way to install PostgreSQL Extensions
PostgreSQL Is Eating the Database World through the power of extensibility. With 400 extensions powering PostgreSQL, we may not say it’s invincible, but it’s definitely getting much closer.
I believe the PostgreSQL community has reached a consensus on the importance of extensions. So the real question now becomes: “What should we do about it?”
What’s the primary problem with PostgreSQL extensions? In my opinion, it’s their accessibility. Extensions are useless if most users can’t easily install and enable them. But it’s not that easy.
Even the largest cloud postgres vendors are struggling with this. They have some inherent limitations (multi-tenancy, security, licensing) that make it hard for them to fully address this issue.
So here’s my plan, I’ve created a repository that hosts 400 of the most capable extensions in the PostgreSQL ecosystem, available as RPM / DEB packages on mainstream Linux OS distros. And the goal is to take PostgreSQL one solid step closer to becoming the all-powerful database and achieve the great alignment between the Debian and EL OS ecosystems.
The status quo
The PostgreSQL ecosystem is rich with extensions, but how do you actually install and use them? This initial hurdle becomes a roadblock for many. There are some existing solutions:
PGXN says, “You can download and compile extensions on the fly with pgxnclient
.”
Tembo says, “We have prepared pre-configured extension stack as Docker images.”
StackGres & Omnigres says, “We download .so
files on the fly.” All solid ideas.
While based on my experience, the vast majority of users still rely on their operating system’s package manager to install PG extensions. On-the-fly compilation and downloading shared libraries might not be a viable option for production env. Since many DB setups don’t have internet access or a proper toolchain ready.
In the meantime, Existing OS package managers like yum
/dnf
/apt
already solve issues like dependency resolution, upgrades, and version management well.
There’s no need to reinvent the wheel or disrupt existing standards. So the real question is: Who’s going to package these extensions into ready-to-use software?
PGDG has already made a fantastic effort with official YUM and APT repositories. In addition to the 70 built-in Contrib extensions bundled with PostgreSQL,the PGDG YUM repo offers 128 RPM extensions, while the APT repo offers 104 DEB extensions. These extensions are compiled and packaged in the same environment as the PostgreSQL kernel, making them easy to install alongside the PostgreSQL binary packages. In fact, even most PostgreSQL Docker images rely on the PGDG repo to install extensions.
I’m deeply grateful for Devrim’s maintenance of the PGDG YUM repo and Christoph’s work with the APT repo. Their efforts to make PostgreSQL installation and extension management seamless are incredibly valuable. But as a distribution creator myself, I’ve encountered some challenges with PostgreSQL extension distribution.
What’s the challenge?
The first major issue facing extension users is Alignment.
In the two primary Linux distro camps — Debian and EL — there’s a significant number of PostgreSQL extensions. Excluding the 70 built-in Contrib extensions bundled with PostgreSQL, the YUM repo offers 128 extensions, and the APT repo provides 104.
However, when we dig deeper, we see that alignment between the two repos is not ideal. The combined total of extensions across both repos is 153, but the overlap is just 79. That means only half of the extensions are available in both ecosystems!
Only half of the extensions are available in both EL and Debian ecosystems!
Next, we run into further alignment issues within each ecosystem itself. The availability of extensions can vary between different major OS versions.
For instance, pljava
, sequential_uuids
, and firebird_fdw
are only available in EL9, but not in EL8. Similarly, rdkit
is available in Ubuntu 22+ / Debian 12+, but not in Ubuntu 20 / Debian 11.
There’s also the issue of architecture support. For example, citus
does not provide arm64
packages in the Debian repo.
And then we have alignment issues across different PostgreSQL major versions. Some extensions won’t compile on older PostgreSQL versions, while others won’t work on newer ones. Some extensions are only available for specific PostgreSQL versions in certain distributions, and so on.
These alignment issues lead to a significant number of permutations. For example, if we consider five mainstream OS distributions (el8, el9, debian12, ubuntu22, ubuntu24),
two CPU architectures (x86_64
and arm64
), and six PostgreSQL major versions (12–17), that’s 60-70 RPM/DEB packages per extension—just for one extension!
On top of alignment, there’s the problem of completeness. PGXN lists over 375 extensions, but the PostgreSQL ecosystem could have as many as 1,000+. The PGDG repos, however, contain only about one-tenth of them.
There are also several powerful new Rust-based extensions that PGDG doesn’t include, such as pg_graphql
, pg_jsonschema
, and wrappers
for self-hosting Supabase;
pg_search
as an Elasticsearch alternative; and pg_analytics
, pg_parquet
, pg_mooncake
for OLAP processing. The reason? They are too slow to compile…
What’s the solution?
Over the past six months, I’ve focused on consolidating the PostgreSQL extension ecosystem. Recently, I reached a milestone I’m quite happy with. I’ve created a PG YUM/APT repository with a catalog of 400available PostgreSQL extensions.
Here are some key stats for the repo: It hosts 400 extensions in total. Excluding the 70 built-in extensions that come with PostgreSQL, this leaves 270 third-party extensions. Of these, about half are maintained by the official PGDG repos (126 RPM, 102 DEB). The other half (131 RPM, 143DEB) are maintained, fixed, compiled, packaged, and distributed by myself.
OS \ Entry | All | PGDG | PIGSTY | CONTRIB | MISC | MISS | PG17 | PG16 | PG15 | PG14 | PG13 | PG12 |
---|---|---|---|---|---|---|---|---|---|---|---|---|
RPM | 334 | 115 | 143 | 70 | 4 | 6 | 301 | 330 | 333 | 319 | 307 | 294 |
DEB | 326 | 104 | 144 | 70 | 4 | 14 | 302 | 322 | 325 | 316 | 303 | 293 |
For each extension, I’ve built versions for the 6 major PostgreSQL versions (12–17) across five popular Linux distributions: EL8, EL9, Ubuntu 22.04, Ubuntu 24.04, and Debian 12. I’ve also provided some limited support for older OS versions like EL7, Debian 11, and Ubuntu 20.04.
This repository also addresses most of the alignment issue. Initially, there were extensions in the APT and YUM repos that were unique to each, but I’ve worked to port as many of these unique extensions to the other ecosystem. Now, only 7 APT extensions are missing from the YUM repo, and 16 extensions are missing in APT—just 6% of the total. Many missing PGDG extensions have also been resolved.
I’ve created a comprehensive directory listing all supported extensions, with detailed info, dependency installation instructions, and other important notes.
I hope this repository can serve as the ultimate solution to the frustration users face when extensions are difficult to find, compile, or install.
How to use this repo?
Now, for a quick plug — what’s the easiest way to install and use these extensions?
The simplest option is to use the OSS PostgreSQL distribution: Pigsty. The repo is autoconfigured by default, so all you need to do is declare them in the config inventory.
For example, the self-hosting supabase template requires extensions that aren’t available in the PGDG repo. You can simply download, install, preload, config and create extensions by referring to their names.
all:
children:
pg-meta:
hosts: { 10.10.10.10: { pg_seq: 1, pg_role: primary } }
vars:
pg_cluster: pg-meta
# INSTALL EXTENSIONS
pg_extensions:
- supabase # essential extensions for supabase
- timescaledb postgis pg_graphql pg_jsonschema wrappers pg_search pg_analytics pg_parquet plv8 duckdb_fdw pg_cron pg_timetable pgqr
- supautils pg_plan_filter passwordcheck plpgsql_check pgaudit pgsodium pg_vault pgjwt pg_ecdsa pg_session_jwt index_advisor
- pgvector pgvectorscale pg_summarize pg_tiktoken pg_tle pg_stat_monitor hypopg pg_hint_plan pg_http pg_net pg_smtp_client pg_idkit
# LOAD EXTENSIONS
pg_libs: 'pg_stat_statements, plpgsql, plpgsql_check, pg_cron, pg_net, timescaledb, auto_explain, pg_tle, plan_filter'
# CONFIG EXTENSIONS
pg_parameters:
cron.database_name: postgres
pgsodium.enable_event_trigger: off
# CREATE EXTENSIONS
pg_databases:
- name: postgres
baseline: supabase.sql
schemas: [ extensions ,auth ,realtime ,storage ,graphql_public ,supabase_functions ,_analytics ,_realtime ]
extensions:
- { name: pgcrypto ,schema: extensions }
- { name: pg_net ,schema: extensions }
- { name: pgjwt ,schema: extensions }
- { name: uuid-ossp ,schema: extensions }
- { name: pgsodium }
- { name: supabase_vault }
- { name: pg_graphql }
- { name: pg_jsonschema }
- { name: wrappers }
- { name: http }
- { name: pg_cron }
- { name: timescaledb }
- { name: pg_tle }
- { name: vector }
vars:
pg_version: 17
# DOWNLOAD EXTENSIONS
repo_extra_packages:
- pgsql-main
- supabase # essential extensions for supabase
- timescaledb postgis pg_graphql pg_jsonschema wrappers pg_search pg_analytics pg_parquet plv8 duckdb_fdw pg_cron pg_timetable pgqr
- supautils pg_plan_filter passwordcheck plpgsql_check pgaudit pgsodium pg_vault pgjwt pg_ecdsa pg_session_jwt index_advisor
- pgvector pgvectorscale pg_summarize pg_tiktoken pg_tle pg_stat_monitor hypopg pg_hint_plan pg_http pg_net pg_smtp_client pg_idkit
To simply add extensions to existing clusters:
./infra.yml -t repo_build -e '{"repo_packages":[citus]}' # download
./pgsql.yml -t pg_extension -e '{"pg_extensions": ["citus"]}' # install
Through this repo was meant to be used with Pigsty, But it is not mandatory. You can still enable this repository on any EL/Debian/Ubuntu system with a simple one-liner in the shell:
APT Repo
For Ubuntu 22.04 & Debian 12 or any compatible platforms, use the following commands to add the APT repo:
curl -fsSL https://repo.pigsty.io/key | sudo gpg --dearmor -o /etc/apt/keyrings/pigsty.gpg
sudo tee /etc/apt/sources.list.d/pigsty-io.list > /dev/null <<EOF
deb [signed-by=/etc/apt/keyrings/pigsty.gpg] https://repo.pigsty.io/apt/infra generic main
deb [signed-by=/etc/apt/keyrings/pigsty.gpg] https://repo.pigsty.io/apt/pgsql/$(lsb_release -cs) $(lsb_release -cs) main
EOF
sudo apt update
YUM Repo
For EL 8/9 and compatible platforms, use the following commands to add the YUM repo:
curl -fsSL https://repo.pigsty.io/key | sudo tee /etc/pki/rpm-gpg/RPM-GPG-KEY-pigsty >/dev/null # add gpg key
curl -fsSL https://repo.pigsty.io/yum/repo | sudo tee /etc/yum.repos.d/pigsty.repo >/dev/null # add repo file
sudo yum makecache
What’s in this repo?
In this repo, all the extensions are categorized into one of the 15 categories: TIME, GIS, RAG, FTS, OLAP, FEAT, LANG, TYPE, FUNC, ADMIN, STAT, SEC, FDW, SIM, ETL, as shown below.
TIME: timescaledb
timescaledb_toolkit
timeseries
periods
temporal_tables
emaj
table_version
pg_cron
pg_later
pg_background
GIS: postgis
postgis_topology
postgis_raster
postgis_sfcgal
postgis_tiger_geocoder
address_standardizer
address_standardizer_data_us
pgrouting
pointcloud
pointcloud_postgis
h3
h3_postgis
q3c
ogr_fdw
geoip
pg_polyline
pg_geohash
mobilitydb
earthdistance
RAG: vector
vectorscale
vectorize
pg_similarity
smlar
pg_summarize
pg_tiktoken
pgml
pg4ml
FTS: pg_search
pg_bigm
zhparser
hunspell_cs_cz
hunspell_de_de
hunspell_en_us
hunspell_fr
hunspell_ne_np
hunspell_nl_nl
hunspell_nn_no
hunspell_pt_pt
hunspell_ru_ru
hunspell_ru_ru_aot
fuzzystrmatch
pg_trgm
OLAP: citus
citus_columnar
columnar
pg_analytics
pg_duckdb
pg_mooncake
duckdb_fdw
pg_parquet
pg_fkpart
pg_partman
plproxy
pg_strom
tablefunc
FEAT: age
hll
rum
pg_graphql
pg_jsonschema
jsquery
pg_hint_plan
hypopg
index_advisor
plan_filter
imgsmlr
pg_ivm
pgmq
pgq
pg_cardano
rdkit
bloom
LANG: pg_tle
plv8
pllua
hstore_pllua
plluau
hstore_plluau
plprql
pldbgapi
plpgsql_check
plprofiler
plsh
pljava
plr
pgtap
faker
dbt2
pltcl
pltclu
plperl
bool_plperl
hstore_plperl
jsonb_plperl
plperlu
bool_plperlu
jsonb_plperlu
hstore_plperlu
plpgsql
plpython3u
jsonb_plpython3u
ltree_plpython3u
hstore_plpython3u
TYPE: prefix
semver
unit
md5hash
asn1oid
roaringbitmap
pgfaceting
pg_sphere
country
currency
pgmp
numeral
pg_rational
uint
uint128
ip4r
uri
pgemailaddr
acl
debversion
pg_rrule
timestamp9
chkpass
isn
seg
cube
ltree
hstore
citext
xml2
FUNC: topn
gzip
zstd
http
pg_net
pg_smtp_client
pg_html5_email_address
pgsql_tweaks
pg_extra_time
timeit
count_distinct
extra_window_functions
first_last_agg
tdigest
aggs_for_vecs
aggs_for_arrays
arraymath
quantile
lower_quantile
pg_idkit
pg_uuidv7
permuteseq
pg_hashids
sequential_uuids
pg_math
random
base36
base62
pg_base58
floatvec
financial
pgjwt
pg_hashlib
shacrypt
cryptint
pguecc
pgpcre
icu_ext
pgqr
envvar
pg_protobuf
url_encode
refint
autoinc
insert_username
moddatetime
tsm_system_time
dict_xsyn
tsm_system_rows
tcn
uuid-ossp
btree_gist
btree_gin
intarray
intagg
dict_int
unaccent
ADMIN: pg_repack
pg_squeeze
pg_dirtyread
pgfincore
pgdd
ddlx
prioritize
pg_checksums
pg_readonly
safeupdate
pg_permissions
pgautofailover
pg_catcheck
pre_prepare
pgcozy
pg_orphaned
pg_crash
pg_cheat_funcs
pg_savior
table_log
pg_fio
pgpool_adm
pgpool_recovery
pgpool_regclass
pgagent
vacuumlo
pg_prewarm
oid2name
lo
basic_archive
basebackup_to_shell
old_snapshot
adminpack
amcheck
pg_surgery
STAT: pg_profile
pg_show_plans
pg_stat_kcache
pg_stat_monitor
pg_qualstats
pg_store_plans
pg_track_settings
pg_wait_sampling
system_stats
meta
pgnodemx
pg_proctab
pg_sqlog
bgw_replstatus
pgmeminfo
toastinfo
explain_ui
pg_relusage
pg_top
pagevis
powa
pageinspect
pgrowlocks
sslinfo
pg_buffercache
pg_walinspect
pg_freespacemap
pg_visibility
pgstattuple
auto_explain
pg_stat_statements
SEC: passwordcheck_cracklib
supautils
pgsodium
supabase_vault
pg_session_jwt
anon
pg_tde
pgsmcrypto
pgaudit
pgauditlogtofile
pg_auth_mon
credcheck
pgcryptokey
pg_jobmon
logerrors
login_hook
set_user
pg_snakeoil
pgextwlist
pg_auditor
sslutils
noset
sepgsql
auth_delay
pgcrypto
passwordcheck
FDW: wrappers
multicorn
odbc_fdw
jdbc_fdw
mysql_fdw
oracle_fdw
tds_fdw
db2_fdw
sqlite_fdw
pgbouncer_fdw
mongo_fdw
redis_fdw
redis
kafka_fdw
hdfs_fdw
firebird_fdw
aws_s3
log_fdw
dblink
file_fdw
postgres_fdw
SIM: orafce
pgtt
session_variable
pg_statement_rollback
pg_dbms_metadata
pg_dbms_lock
pg_dbms_job
babelfishpg_common
babelfishpg_tsql
babelfishpg_tds
babelfishpg_money
pgmemcache
ETL: pglogical
pglogical_origin
pglogical_ticker
pgl_ddl_deploy
pg_failover_slots
wal2json
wal2mongo
decoderbufs
decoder_raw
test_decoding
mimeo
repmgr
pg_fact_loader
pg_bulkload
Check ext.pigsty.io for all the details.
Some Thoughts
Each major PostgreSQL version introduces changes, making the maintenance of 140+ extension packages a bit of a beast.
Especially when some extension authors haven’t updated their work in years. In these cases, you often have no choice but to take matters into your own hands. I’ve personally fixed several extensions and ensured they support the latest PostgreSQL major versions. For those authors I could reach, I’ve submitted numerous PRs and issues to keep things moving forward.
Back to the point: my goal with this repo is to establish a standard for PostgreSQL extension installation and distribution, solving the distribution challenges that have long troubles the users.
A recent milestone is that, the popular open-source PostgreSQL HA cluster project postgresql_cluster, has made this extension repository the default upstream for PG extension installation.
Currently, this repository (repo.pigsty.io) is hosted on Cloudflare. In the past month, the repo and its mirrors have served about 300GB of downloads. Given that most extensions are just a few KB to a few MB, that amounts to nearly a million downloads per month. Since Cloudflare doesn’t charge for traffic, I can confidently commit to keeping this repository completely free & under active maintenance for the foreseeable future, as long as cloudflare doesn’t charge me too much.
I believe my work can help PostgreSQL users worldwide and contribute to the thriving PostgreSQL ecosystem. I hope it proves useful to you as well. Enjoy PostgreSQL!
Self-Hosting Dify with PG, PGVector, and Pigsty
Dify – The Innovation Engine for GenAI Applications
Dify is an open-source LLM app development platform. Orchestrate LLM apps from agents to complex AI workflows, with an RAG engine. Which claims to be more production-ready than LangChain.
Of course, a workflow orchestration software like this needs a database underneath — Dify uses PostgreSQL for meta data storage, as well as Redis for caching and a dedicated vector database. You can pull the Docker images and play locally, but for production deployment, this setup won’t suffice — there’s no HA, backup, PITR, monitoring, and many other things.
Fortunately, Pigsty provides a battery-include production-grade highly available PostgreSQL cluster, along with the Redis and S3 (MinIO) capabilities that Dify needs, as well as Nginx to expose the Web service, making it the perfect companion for Dify.
Off-load the stateful part to Pigsty, you only need to pull up the stateless blue circle part with a simple
docker compose up
.
BTW, I have to criticize the design of the Dify template. Since the metadata is already stored in PostgreSQL, why not add pgvector
to use it as a vector database? What’s even more baffling is that pgvector
is a separate image and container. Why not just use a PG image with pgvector
included?
Dify “supports” a bunch of flashy vector databases, but since PostgreSQL is already chosen, using pgvector
as the default vector database is the natural choice. Similarly, I think the Dify team should consider removing Redis. Celery task queues can use PostgreSQL as backend storage, so having multiple databases is unnecessary. Entities should not be multiplied without necessity.
Therefore, the Pigsty-provided Dify Docker Compose template has made some adjustments to the official example. It removes the db
and redis
database images, using instances managed by Pigsty. The vector database is fixed to use pgvector
, reusing the same PostgreSQL instance.
In the end, the architecture is simplified to three stateless containers: dify-api
, dify-web
, and dify-worker
, which can be created and destroyed at will. There are also two optional containers, ssrf_proxy
and nginx
, for providing proxy and some security features.
There’s a bit of state management left with file system volumes, storing things like private keys. Regular backups are sufficient.
Reference:
Pigsty Preparation
Let’s take the single-node installation of Pigsty as an example. Suppose you have a machine with the IP address 10.10.10.10
and already pigsty installed.
We need to define the database clusters required in the Pigsty configuration file pigsty.yml
.
Here, we define a cluster named pg-meta
, which includes a superuser named dbuser_dify
(the implementation is a bit rough as the Migration script executes CREATE EXTENSION
which require dbsu privilege for now),
And there’s a database named dify
with the pgvector
extension installed, and a specific firewall rule allowing users to access the database from anywhere using a password (you can also restrict it to a more precise range, such as the Docker subnet 172.0.0.0/8
).
Additionally, a standard single-instance Redis cluster redis-dify
with the password redis.dify
is defined.
pg-meta:
hosts: { 10.10.10.10: { pg_seq: 1, pg_role: primary } }
vars:
pg_cluster: pg-meta
pg_users: [ { name: dbuser_dify ,password: DBUser.Dify ,superuser: true ,pgbouncer: true ,roles: [ dbrole_admin ] } ]
pg_databases: [ { name: dify, owner: dbuser_dify, extensions: [ { name: pgvector } ] } ]
pg_hba_rules: [ { user: dbuser_dify , db: all ,addr: world ,auth: pwd ,title: 'allow dify user world pwd access' } ]
redis-dify:
hosts: { 10.10.10.10: { redis_node: 1 , redis_instances: { 6379: { } } } }
vars: { redis_cluster: redis-dify ,redis_password: 'redis.dify' ,redis_max_memory: 64MB }
For demonstration purposes, we use single-instance configurations. You can refer to the Pigsty documentation to deploy high availability PG and Redis clusters. After defining the clusters, use the following commands to create the PG and Redis clusters:
bin/pgsql-add pg-meta # create the dify database cluster
bin/redis-add redis-dify # create redis cluster
Alternatively, you can define a new business user and business database on an existing PostgreSQL cluster, such as pg-meta
, and create them with the following commands:
bin/pgsql-user pg-meta dbuser_dify # create dify biz user
bin/pgsql-db pg-meta dify # create dify biz database
You should be able to access PostgreSQL and Redis with the following connection strings, adjusting the connection information as needed:
psql postgres://dbuser_dify:[email protected]:5432/dify -c 'SELECT 1'
redis-cli -u redis://[email protected]:6379/0 ping
Once you confirm these connection strings are working, you’re all set to start deploying Dify.
For demonstration purposes, we’re using direct IP connections. For a multi-node high availability PG cluster, please refer to the service access section.
The above assumes you are already a Pigsty user familiar with deploying PostgreSQL and Redis clusters. You can skip the next section and proceed to see how to configure Dify.
Starting from Scratch
If you’re already familiar with setting up Pigsty, feel free to skip this section.
Prepare a fresh Linux x86_64 node that runs compatible OS, then run as a sudo-able user:
curl -fsSL https://repo.pigsty.io/get | bash
It will download Pigsty source to your home, then perform configure and install to finish the installation.
cd ~/pigsty # get pigsty source and entering dir
./bootstrap # download bootstrap pkgs & ansible [optional]
./configure # pre-check and config templating [optional]
# change pigsty.yml, adding those cluster definitions above into all.children
./install.yml # install pigsty according to pigsty.yml
You should insert the above PostgreSQL cluster and Redis cluster definitions into the pigsty.yml
file, then run install.yml
to complete the installation.
Redis Deploy
Pigsty will not deploy redis in install.yml
, so you have to run redis.yml
playbook to install Redis explicitly:
./redis.yml
Docker Deploy
Pigsty will not deploy Docker by default, so you need to install Docker with the docker.yml
playbook.
./docker.yml
Dify Confiugration
You can configure dify in the .env
file:
All parameters are self-explanatory and filled in with default values that work directly in the Pigsty sandbox env. Fill in the database connection information according to your actual conf, consistent with the PG/Redis cluster configuration above.
Changing the SECRET_KEY
field is recommended. You can generate a strong key with openssl rand -base64 42
:
# meta parameter
DIFY_PORT=8001 # expose dify nginx service with port 8001 by default
LOG_LEVEL=INFO # The log level for the application. Supported values are `DEBUG`, `INFO`, `WARNING`, `ERROR`, `CRITICAL`
SECRET_KEY=sk-9f73s3ljTXVcMT3Blb3ljTqtsKiGHXVcMT3BlbkFJLK7U # A secret key for signing and encryption, gen with `openssl rand -base64 42`
# postgres credential
PG_USERNAME=dbuser_dify
PG_PASSWORD=DBUser.Dify
PG_HOST=10.10.10.10
PG_PORT=5432
PG_DATABASE=dify
# redis credential
REDIS_HOST=10.10.10.10
REDIS_PORT=6379
REDIS_USERNAME=''
REDIS_PASSWORD=redis.dify
# minio/s3 [OPTIONAL] when STORAGE_TYPE=s3
STORAGE_TYPE=local
S3_ENDPOINT='https://sss.pigsty'
S3_BUCKET_NAME='infra'
S3_ACCESS_KEY='dba'
S3_SECRET_KEY='S3User.DBA'
S3_REGION='us-east-1'
Now we can pull up dify with docker compose:
cd pigsty/app/dify && make up
Expose Dify Service via Nginx
Dify expose web/api via its own nginx through port 80 by default, while pigsty uses port 80 for its own Nginx. T
herefore, we expose Dify via port 8001
by default, and use Pigsty’s Nginx to forward to this port.
Change infra_portal
in pigsty.yml
, with the new dify
line:
infra_portal: # domain names and upstream servers
home : { domain: h.pigsty }
grafana : { domain: g.pigsty ,endpoint: "${admin_ip}:3000" , websocket: true }
prometheus : { domain: p.pigsty ,endpoint: "${admin_ip}:9090" }
alertmanager : { domain: a.pigsty ,endpoint: "${admin_ip}:9093" }
blackbox : { endpoint: "${admin_ip}:9115" }
loki : { endpoint: "${admin_ip}:3100" }
dify : { domain: dify.pigsty ,endpoint: "10.10.10.10:8001", websocket: true }
Then expose dify web service via Pigsty’s Nginx server:
./infra.yml -t nginx
Don’t forget to add dify.pigsty
to your DNS or local /etc/hosts
/ C:\Windows\System32\drivers\etc\hosts
to access via domain name.
PGCon.Dev 2024, The conf that shutdown PG for a week
PGCon.Dev, once known as PGCon—the annual must-attend gathering for PostgreSQL hackers and key forum for its future direction, has been held in Ottawa since its inception in 2007.
This year marks a new chapter as the original organizer, Dan, hands over the reins to a new team, and the event moves to SFU’s Harbour Centre in Vancouver, kicking off a new era with grandeur.
How engaging was this event? Peter Eisentraut, member of the PostgreSQL core team, noted that during PGCon.Dev, there were no code commits to PostgreSQL – resulting in the longest pause in twenty years, a whopping week! a historic coding ceasefire! Why? Because all the developers were at the conference!
Considering the last few interruptions that occurred in the early days of the project twenty years ago,
I’ve been embracing PostgreSQL for a decade, but attending a global PG Hacker conference in person was a first for me, and I’m immensely grateful for the organizer’s efforts. PGCon.Dev 2024 wrapped up on May 31st, though this post comes a bit delayed as I’ve been exploring Vancouver and Banff National Park ;)
Day Zero: Extension Summit
Day zero is for leadership meetings, and I’ve signed up for the afternoon’s Extension Ecosystem Summit.
Maybe this summit is somewhat subtly related to my recent post, “Postgres is eating the database world,” highlighting PostgreSQL’s thriving extension ecosystem as a unique and critical success factor and drawing the community’s attention.
I participated in David Wheeler’s Binary Packing session along with other PostgreSQL community leaders. Despite some hesitation to new standards like PGXN v2 from current RPM/APT maintainers. In the latter half of the summit, I attended a session led by Yurii Rashkovskii, discussing extension directory structures, metadata, naming conflicts, version control, and binary distribution ideas.
Prior to this summit, the PostgreSQL community had held six mini-summits discussing these topics intensely, with visions for the extension ecosystem’s future development shared by various speakers. Recordings of these sessions are available on YouTube.
And after the summit, I had a chance to chat with Devrim, the RPM maintainer, about extension packing, which was quite enlightening.
“Keith Fan Group” – from Devrim on Extension Summit
Day One: Brilliant Talks and Bar Social
The core of PGCon.Dev lies in its sessions. Unlike some China domestic conferences with mundane product pitches or irrelevant tech details, PGCon.Dev presentations are genuinely engaging and substantive. The official program kicked off on May 29th, after a day of closed-door leadership meetings and the Ecosystem Summit on the 28th.
The opening was co-hosted by Jonathan Katz, 1 of the 7 core PostgreSQL team members and a chief product manager at AWS RDS, and Melanie Plageman, a recent PG committer from Microsoft. A highlight was when Andres Freund, the developer who uncovered the famous xz
backdoor, was celebrated as a superhero on stage.
Following the opening, the regular session tracks began. Although conference videos aren’t out yet, I’m confident they’ll “soon” be available on YouTube. Most sessions had three tracks running simultaneously; here are some highlights I chose to attend.
Pushing the Boundaries of PG Extensions
Yurii’s talk, “Pushing the Boundaries of PG Extensions,” tackled what kind of extension APIs PostgreSQL should offer. PostgreSQL boasts robust extensibility, but the current extension API set is decades old, from the 9.x era. Yurii’s proposal aims to address issues with the existing extension mechanisms. Challenges such as installing multiple versions of an extension simultaneously, avoiding database restarts post-extension installations, managing extensions as seamlessly as data, and handling dependencies among extensions were discussed.
Yurii and Viggy, founders of Omnigres, aim to transform PostgreSQL into a full-fledged application development platform, including hosting HTTP servers directly within the database. They designed a new extension API and management system for PostgreSQL to achieve this. Their innovative improvements represent the forefront of exploration into PostgreSQL’s core extension mechanisms.
I had a great conversation with Viggy and Yurii. Yurii walked me through compiling and installing Omni. I plan to support the Omni extension series in the next version of Pigsty, making this powerful application development framework plug-and-play.
Anarchy in DBMS
Abigale Kim from CMU, under the mentorship of celebrity professor Andy Pavlo, delivered the talk “Anarchy in the Database—A Survey and Evaluation of DBMS Extensibility.” This topic intrigued me since Pigsty’s primary value proposition is about PostgreSQL’s extensibility.
Kim’s research revealed interesting insights: PostgreSQL is the most extensible DBMS, supporting 9 out of 10 extensibility points, closely followed by DuckDB. With over 375+ available extensions, PostgreSQL significantly outpaces other databases.
Kim’s quantitative analysis of compatibility levels among these extensions resulted in a compatibility matrix, unveiling conflicts—most notably, powerful extensions like TimescaleDB and Citus are prone to clashes. This information is very valuable for users and distribution maintainers. Read the detailed study.
I joked with Kim that — now I could brag about PostgreSQL’s extensibility with her research data.
How PostgreSQL is Misused and Abused
The first-afternoon session featured Karen Jex from CrunchyData, an unusual perspective from a user — and a female DBA. Karen shared common blunders by PostgreSQL beginners. While I knew all of what was discussed, it reaffirmed that beginners worldwide make similar mistakes — an enlightening perspective for PG Hackers, who found the session quite engaging.
PostgreSQL and the AI Ecosystem
The second-afternoon session by Bruce Momjian, co-founder of the PGDG and a core committee member from the start, was unexpectedly about using PostgreSQL’s multi-dimensional arrays and queries to implement neural network inference and training.
Haha, some ArgParser code. I see it
During the lunch, Bruce explained that Jonathan Katz needed a topic to introduce the vector database extension PGVector in the PostgreSQL ecosystem, so Bruce was roped in to “fill the gap.” Check out Bruce’s presentation.
PB-Level PostgreSQL Deployments
The third afternoon session by Chris Travers discussed their transition from using ElasticSearch for data storage—with a poor experience and high maintenance for 1PB over 30 days retention, to a horizontally scaled PostgreSQL cluster perfectly handling 10PB of data. Normally, PostgreSQL comfort levels on a single machine range from several dozen to a few hundred TB. Deployments at the PB scale, especially at 10PB, even within a horizontally scaled cluster, are exceptionally rare. While the practice itself is standard—partitioning and sharding—the scale of data managed is truly impressive.
Highlight: When Hardware and Database Collide
Undoubtedly, the standout presentation of the event, Margo Seltzer’s talk “When Hardware and Database Collide” was not only the most passionate and compelling talk I’ve attended live but also a highlight across all conferences.
Professor Margo Seltzer, formerly of Harvard and now at UBC, a member of the National Academy of Engineering and the creator of BerkeleyDB, delivered a powerful discourse on the core challenges facing databases today. She pinpointed that the bottleneck for databases has shifted from disk I/O to main memory speed. Emerging hardware technologies like HBM and CXL could be the solution, posing new challenges for PostgreSQL hackers to tackle.
This was a refreshing divergence from China’s typically monotonous academic talks, leaving a profound impact and inspiration. Once the conference video is released, I highly recommend checking out her energizing presentation.
WetBar Social
Following Margo’s session, the official Social Event took place at Rogue Kitchen & Wetbar, just a street away from the venue at Waterfront Station, boasting views of the Pacific and iconic Vancouver landmarks.
The informal setting was perfect for engaging with new and old peers. Conversations with notable figures like Devrim, Tomasz, Yurii, and Keith were particularly enriching. As an RPM maintainer, I had an extensive and fruitful discussion with Devrim, resolving many longstanding queries.
The atmosphere was warm and familiar, with many reconnecting after long periods. A couple of beers in, conversations flowed even more freely among fellow PostgreSQL enthusiasts. The event concluded with an invitation from Melanie for a board game session, which I regretfully declined due to my limited English in such interactive settings.
Day 2: Debate, Lunch, and Lighting Talks
Multi-Threading Postgres
The warmth from the previous night’s socializing carried over into the next day, marked by the eagerly anticipated session on “Multi-threaded PostgreSQL,” which was packed to capacity. The discussion, initiated by Heikki, centered on the pros and cons of PostgreSQL’s process and threading models, along with detailed implementation plans and current progress.
The threading model promises numerous benefits: cheaper connections (akin to a built-in connection pool), shared relation and plan caches, dynamic adjustment of shared memory, config changes without restarts, more aggressive Vacuum operations, runtime Explain Analyze, and easier memory usage limits per connection. However, there’s significant opposition, maybe led by Tom Lane, concerned about potential bugs, loss of isolation benefits from the multi-process model, and extensive incompatibilities requiring many extensions to be rewritten.
Heikki laid out a detailed plan to transition to the threading model over five to seven years, aiming for a seamless shift without intermediate states. Intriguingly, he cited Tom Lane’s critical comment in his presentation:
For the record, I think this will be a disaster. There is far too much code that will get broken, largely silently, and much of it is not under our control. – regards, tom lane
Although Tom Lane smiled benignly without voicing any objections, the strongest dissent at the conference came not from him but from an extension maintainer. The elder developer, who maintained several extensions, raised concerns about compatibility, specifically regarding memory allocation and usage. Heikki suggested that extension authors should adapt their work to a new model during a transition grace period of about five years. This suggestion visibly upset the maintainer, who left the meeting in anger.
Given the proposed threading model’s significant impact on the existing extension ecosystem, I’m skeptical about this change. At the conference, I consulted on the threading model with Heikki, Tom Lane, and other hackers. The community’s overall stance is one of curious & cautious observation. So far, the only progress is in PG 17, where the fork-exec-related code has been refactored and global variables marked for future modifications. Any real implementation would likely not occur until at least PG 20+.
Hallway Track
The sessions on the second day were slightly less intense than the first, so many attendees chose the “Hallway Track”—engaging in conversations in the corridors and lobby. I’m usually not great at networking as an introvert, but the vibrant atmosphere quickly drew me in. Eye contact alone was enough to spark conversations, like triggering NPC dialogue in an RPG. I also managed to subtly promote Pigsty to every corner of the PG community.
Despite being a first-timer at PGCon.Dev, I was surprised by the recognition and attention I received, largely thanks to the widely read article, “PostgreSQL is eating the Database world.” Many recognized me by my badge Vonng / Pigsty.
A simple yet effective networking trick is never to underestimate small gifts’ effect. I handed out gold-plated Slonik pins, PostgreSQL’s mascot, which became a coveted item at the conference. Everyone who talked with me received one, and those who didn’t have one were left asking where to get one. LOL
Anyway, I’m glad to have made many new friends and connections.
Multinational Community Lunch
As for lunch, HighGo hosted key participants from the American, European, Japanese, and Chinese PostgreSQL communities at a Cantonese restaurant in Vancouver. The conversation ranged from serious technical discussions to lighter topics. I’ve made acquaintance with Tatsuro Yamada, who gives a talk, “Advice is seldom welcome but efficacious”, and Kyotaro Horiguchi, a core contributor to PostgreSQL known for his work on WAL replication and multibyte string processing and the author of pg_hint_plan.
Another major contributor to the PostgreSQL community, Mark Wong organizes PGUS and has developed a series of PostgreSQL monitoring extensions. He also manages community merchandise like contributor coins, shirts, and stickers. He even handcrafted a charming yarn elephant mascot, which was so beloved that one was sneakily “borrowed” at the last PG Conf US.
Bruce, already a familiar face in the PG Chinese community, Andreas Scherbaum from Germany, organizer of the European PG conferences, and Miao Jian, founder of Han Gao, representing the only Chinese database company at PGCon.Dev, all shared insightful stories and discussions about the challenges and nuances of developing databases in their respective regions.
On returning to the conference venue, I had a conversation with Jan Wieck, a PostgreSQL Hackers Emeritus. He shared his story of participating in the PostgreSQL project from the early days and encouraged me to get more involved in the PostgreSQL community, reminding me its future depends on the younger generation.
Making PG Hacking More Inclusive
At PGCon.Dev, a special session on community building chaired by Robert Hass, featured three new PostgreSQL contributors sharing their journey and challenges, notably the barriers for non-native English speakers, timezone differences, and emotionally charged email communications.
Robert emphasized in a post-conference blog his desire to see more developers from India and Japan rise to senior positions within PostgreSQL’s ranks, noting the underrepresentation from these countries despite their significant developer communities.
While we’re at it, I’d really like to see more people from India and Japan in senior positions within the project. We have very large developer communities from both countries, but there is no one from either of those countries on the core team, and they’re also underrepresented in other senior positions. At the risk of picking specific examples to illustrate a general point, there is no one from either country on the infrastructure team or the code of conduct committee. We do have a few committers from those countries, which is very good, and I was pleased to see Amit Kapila on the 2024.pgconf.dev organizing commitee, but, overall, I think we are still not where we should be. Part of getting people involved is making them feel like they are not alone, and part of it is also making them feel like progression is possible. Let’s try harder to do that.
Frankly, the lack of mention of China in discussions about inclusivity at PGCon.Dev, in favor of India and Japan, left a bittersweet taste. But I think China deserves the snub, given its poor international community engagement.
China has hundreds of “domestic/national” databases, many mere forks of PostgreSQL, yet there’s only a single notable Chinese contributor to PostgreSQL is Richard Guo from PieCloudDB, recently promoted to PG Committer. At the conference, the Chinese presence was minimal, summing up to five attendees, including myself. It’s regrettable that China’s understanding and adoption of PostgreSQL lag behind the global standard by about 10-15 years.
I hope my involvement can bootstrap and enhance Chinese participation in the global PostgreSQL ecosystem, making their users, developers, products, and open-source projects more recognized and accepted worldwide.
Lightning Talks
Yesterday’s event closed with a series of lightning talks—5 minutes max per speaker, or you’re out. Concise and punchy, the session wrapped up 11 topics in just 45 minutes. Keith shared improvements to PG Monitor, and Peter Eisentraut discussed SQL standard updates. But from my perspective, the highlight was Devrim Gündüz’s talk on PG RPMs, which lived up to his promise of a “big reveal” made at the bar the previous night, packing a 75-slide presentation into 5 lively minutes.
Speaking of PostgreSQL, despite being open-source, most users rely on official pre-compiled binaries packages rather than building from source. I maintain 34 RPM extensions for Pigsty, my Postgres distribution, but much of the ecosystem, including over a hundred other extensions, is managed by Devrim from the official PGDG repo. His efforts ensure quality for the world’s most advanced and popular database.
Devrim is a fascinating character — a Turkish native living in London, a part-time DJ, and the maintainer of the PGDG RPM repository, sporting a PostgreSQL logo tattoo. After an engaging chat about the PGDG repository, he shared insights on how extensions are added, highlighting the community-driven nature of PGXN and recent popular additions like pgvector
, (which I made the suggestion haha).
Interestingly, with the latest Pigsty v2.7 release, four of my maintained (packaging) extensions (pgsql-http
, pgsql-gzip
, pg_net
, pg_bigm
) were adopted into the PGDG official repository. Devrim admitted to scouring Pigsty’s extension list for good picks, though he humorously dismissed any hopes for my Rust pgrx extensions making the cut, reaffirming his commitment to not blending Go and Rust plugins into the official repository. Our conversation was so enriching that I’ve committed myself to becoming a “PG Extension Hunter,” scouting and recommending new plugins for official inclusion.
Day 3: Unconference
One of the highlights of PGCon.Dev is the Unconference, a self-organized meeting with no predefined agenda, driven by attendee-proposed topics. On day three, Joseph Conway facilitated the session where anyone could pitch topics for discussion, which were then voted on by participants. My proposal for a Built-in Prometheus Metrics Exporter was merged into a broader Observability topic spearheaded by Jeremy.
The top-voted topics were Multithreading (42 votes), Observability (35 votes), and Enhanced Community Engagement (35 votes). Observability features were a major focus, reflecting the community’s priority. I proposed integrating a contrib monitoring extension in PostgreSQL to directly expose metrics via HTTP endpoint, using pg_exporter
as a blueprint but embedded to overcome the limitations of external components, especially during crash recovery scenarios.
There’s a clear focus on observability among the community. As the author of pg_exporter, I proposed developing a first-party monitoring extension. This extension would integrate Prometheus monitoring endpoints directly into PostgreSQL, exposing metrics via HTTP without needing external components.
The rationale for this proposal is straightforward. While pg_exporter
works well, it’s an external component that adds management complexity. Additionally, in scenarios where PostgreSQL is recovering from a crash and cannot accept new connections, external tools struggle to access internal states. An in-kernel extension could seamlessly capture this information.
The suggested implementation involves a background worker process similar to the bgw_replstatus
extension. This process would listen on an additional port to expose monitoring metrics through HTTP, using pg_exporter as a blueprint. Metrics would primarily be defined via a Collector configuration table, except for a few critical system indicators.
This idea garnered attention from several PostgreSQL hackers at the event. Developers from EDB and CloudNativePG are evaluating whether pg_exporter
could be directly integrated into their distributions as part of their monitoring solutions. And finally, an Observability Special Interest Group (SIG) was formed by attendees interested in observability, planning to continue discussions through a mailing list.
Issue: Support for LoongArch Architecture
During the last two days, I have had some discussions with PG Hackers about some Chinese-specific issues.
A notable suggestion was supporting the LoongArch architecture in the PGDG global repository, which was backed by some enthusiastically local chip and OS manufacturers. Despite the interest, Devrim indicated a “No” due to the lack of support for LoongArch in OS Distro used in the PG community, like CentOS 7, Rocky 8/9, and Debian 10/11/12. Tomasz Rybak was more receptive, noting potential future support if LoongArch runs on Debian 13.
In summary, official PG RPMs might not yet support LoongArch, but APT has a chance, contingent on broader OS support for mainstream open-source Linux distributions.
Issue: Server-side Chinese Character Encoding
At the recent conference, Jeremy Schneider presented an insightful talk on collation rules that resonated with me. He highlighted the pitfalls of not using C.UTF8 for collation, a practice I’ve advocated for based on my own research, and which is detailed in his presentation here.
Post-talk, I discussed further with Jeremy and Peter Eisentraut the nuances of character sets in China, especially the challenges posed by the mandatory GB18030 standard, which PostgreSQL can handle on the client side but not the server side. Also, there are some issues about 20 Chinese characters not working on the convert_to
+ gb18030
encoding mapping.
Closing
The event closed with Jonathan Katz and Melanie Plageman wrapping up an exceptional conference that leaves us looking forward to next year’s PGCon.Dev 2025 in Canada, possibly in Vancouver, Toronto, Ottawa, or Montreal.
Inspired by the engagement at this conference, I’m considering presenting on Pigsty or PostgreSQL observability next year.
Notably, following the conference, Pigsty’s international CDN traffic spiked significantly, highlighting the growing global reach of our PostgreSQL distribution, which really made my day.
Pigsty CDN Traffic Growth after PGCon.Dev 2024
Some slides are available on the official site, and some blog posts about PGCon are here.Dev 2024:
Postgres is eating the database world
PostgreSQL isn’t just a simple relational database; it’s a data management framework with the potential to engulf the entire database realm. The trend of “Using Postgres for Everything” is no longer limited to a few elite teams but is becoming a mainstream best practice.
OLAP’s New Challenger
In a 2016 database meetup, I argued that a significant gap in the PostgreSQL ecosystem was the lack of a sufficiently good columnar storage engine for OLAP workloads. While PostgreSQL itself offers lots of analysis features, its performance in full-scale analysis on larger datasets doesn’t quite measure up to dedicated real-time data warehouses.
Consider ClickBench, an analytics performance benchmark, where we’ve documented the performance of PostgreSQL, its ecosystem extensions, and derivative databases. The untuned PostgreSQL performs poorly (x1050), but it can reach (x47) with optimization. Additionally, there are three analysis-related extensions: columnar store Hydra (x42), time-series TimescaleDB (x103), and distributed Citus (x262).
ClickBench c6a.4xlarge, 500gb gp2 results in relative time
This performance can’t be considered bad, especially compared to pure OLTP databases like MySQL and MariaDB (x3065, x19700); however, its third-tier performance is not “good enough,” lagging behind the first-tier OLAP components like Umbra, ClickHouse, Databend, SelectDB (x3~x4) by an order of magnitude. It’s a tough spot - not satisfying enough to use, but too good to discard.
However, the arrival of ParadeDB and DuckDB changed the game!
ParadeDB’s native PG extension pg_analytics achieves second-tier performance (x10), narrowing the gap to the top tier to just 3–4x. Given the additional benefits, this level of performance discrepancy is often acceptable - ACID, freshness and real-time data without ETL, no additional learning curve, no maintenance of separate services, not to mention its ElasticSearch grade full-text search capabilities.
DuckDB focuses on pure OLAP, pushing analysis performance to the extreme (x3.2) — excluding the academically focused, closed-source database Umbra, DuckDB is arguably the fastest for practical OLAP performance. It’s not a PG extension, but PostgreSQL can fully leverage DuckDB’s analysis performance boost as an embedded file database through projects like DuckDB FDW and pg_quack.
The emergence of ParadeDB and DuckDB propels PostgreSQL’s analysis capabilities to the top tier of OLAP, filling the last crucial gap in its analytic performance.
The Pendulum of Database Realm
The distinction between OLTP and OLAP didn’t exist at the inception of databases. The separation of OLAP data warehouses from databases emerged in the 1990s due to traditional OLTP databases struggling to support analytics scenarios’ query patterns and performance demands.
For a long time, best practice in data processing involved using MySQL/PostgreSQL for OLTP workloads and syncing data to specialized OLAP systems like Greenplum, ClickHouse, Doris, Snowflake, etc., through ETL processes.
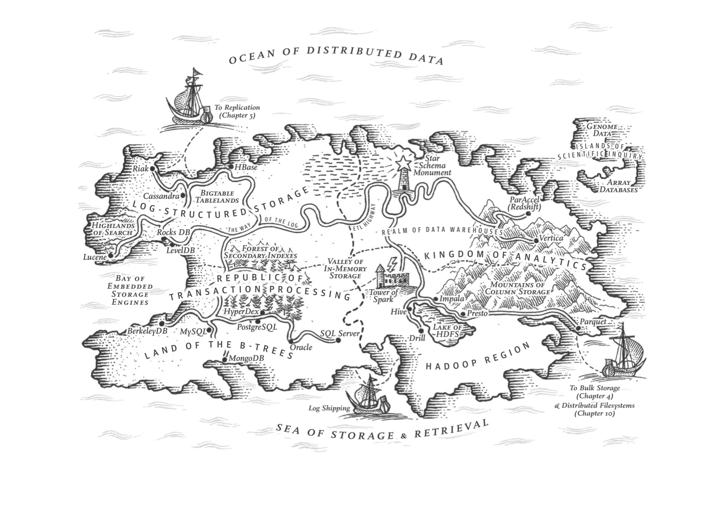
DDIA, Martin Kleppmann, ch3, The republic of OLTP & Kingdom of OLAP
Like many “specialized databases,” the strength of dedicated OLAP systems often lies in performance — achieving 1-3 orders of magnitude improvement over native PG or MySQL. The cost, however, is redundant data, excessive data movement, lack of agreement on data values among distributed components, extra labor expense for specialized skills, extra licensing costs, limited query language power, programmability and extensibility, limited tool integration, poor data integrity and availability compared with a complete DMBS.
However, as the saying goes, “What goes around comes around”. With hardware improving over thirty years following Moore’s Law, performance has increased exponentially while costs have plummeted. In 2024, a single x86 machine can have hundreds of cores (512 vCPU EPYC 9754x2), several TBs of RAM, a single NVMe SSD can hold up to 64TB, and a single all-flash rack can reach 2PB; object storage like S3 offers virtually unlimited storage.
Hardware advancements have solved the data volume and performance issue, while database software developments (PostgreSQL, ParadeDB, DuckDB) have addressed access method challenges. This puts the fundamental assumptions of the analytics sector — the so-called “big data” industry — under scrutiny.
As DuckDB’s manifesto "Big Data is Dead" suggests, the era of big data is over. Most people don’t have that much data, and most data is seldom queried. The frontier of big data recedes as hardware and software evolve, rendering “big data” unnecessary for 99% of scenarios.
If 99% of use cases can now be handled on a single machine with standalone DuckDB or PostgreSQL (and its replicas), what’s the point of using dedicated analytics components? If every smartphone can send and receive texts freely, what’s the point of pagers? (With the caveat that North American hospitals still use pagers, indicating that maybe less than 1% of scenarios might genuinely need “big data.”)
The shift in fundamental assumptions is steering the database world from a phase of diversification back to convergence, from a big bang to a mass extinction. In this process, a new era of unified, multi-modeled, super-converged databases will emerge, reuniting OLTP and OLAP. But who will lead this monumental task of reconsolidating the database field?
PostgreSQL: The Database World Eater
There are a plethora of niches in the database realm: time-series, geospatial, document, search, graph, vector databases, message queues, and object databases. PostgreSQL makes its presence felt across all these domains.
A case in point is the PostGIS extension, which sets the de facto standard in geospatial databases; the TimescaleDB extension awkwardly positions “generic” time-series databases; and the vector extension, PGVector, turns the dedicated vector database niche into a punchline.
This isn’t the first time; we’re witnessing it again in the oldest and largest subdomain: OLAP analytics. But PostgreSQL’s ambition doesn’t stop at OLAP; it’s eyeing the entire database world!
What makes PostgreSQL so capable? Sure, it’s advanced, but so is Oracle; it’s open-source, as is MySQL. PostgreSQL’s edge comes from being both advanced and open-source, allowing it to compete with Oracle/MySQL. But its true uniqueness lies in its extreme extensibility and thriving extension ecosystem.
TimescaleDB survey: what is the main reason you choose to use PostgreSQL
PostgreSQL isn’t just a relational database; it’s a data management framework capable of engulfing the entire database galaxy. Besides being open-source and advanced, its core competitiveness stems from extensibility, i.e., its infra’s reusability and extension’s composability.
The Magic of Extreme Extensibility
PostgreSQL allows users to develop extensions, leveraging the database’s common infra to deliver features at minimal cost. For instance, the vector database extension pgvector, with just several thousand lines of code, is negligible in complexity compared to PostgreSQL’s millions of lines. Yet, this “insignificant” extension achieves complete vector data types and indexing capabilities, outperforming lots of specialized vector databases.
Why? Because pgvector’s creators didn’t need to worry about the database’s general additional complexities: ACID, recovery, backup & PITR, high availability, access control, monitoring, deployment, 3rd-party ecosystem tools, client drivers, etc., which require millions of lines of code to solve well. They only focused on the essential complexity of their problem.
For example, ElasticSearch was developed on the Lucene search library, while the Rust ecosystem has an improved next-gen full-text search library, Tantivy, as a Lucene alternative. ParadeDB only needs to wrap and connect it to PostgreSQL’s interface to offer search services comparable to ElasticSearch. More importantly, it can stand on the shoulders of PostgreSQL, leveraging the entire PG ecosystem’s united strength (e.g., mixed searches with PG Vector) to “unfairly” compete with another dedicated database.
Pigsty has 255 extensions available. And there are 1000+ more in the ecosystem
The extensibility brings another huge advantage: the composability of extensions, allowing different extensions to work together, creating a synergistic effect where 1+1 » 2. For instance, TimescaleDB can be combined with PostGIS for spatio-temporal data support; the BM25 extension for full-text search can be combined with the PGVector extension, providing hybrid search capabilities.
Furthermore, the distributive extension Citus can transparently transform a standalone cluster into a horizontally partitioned distributed database cluster. This capability can be orthogonally combined with other features, making PostGIS a distributed geospatial database, PGVector a distributed vector database, ParadeDB a distributed full-text search database, and so on.
What’s more powerful is that extensions evolve independently, without the cumbersome need for main branch merges and coordination. This allows for scaling — PG’s extensibility lets numerous teams explore database possibilities in parallel, with all extensions being optional, not affecting the core functionality’s reliability. Those features that are mature and robust have the chance to be stably integrated into the main branch.
PostgreSQL achieves both foundational reliability and agile functionality through the magic of extreme extensibility, making it an outlier in the database world and changing the game rules of the database landscape.
Game Changer in the DB Arena
The emergence of PostgreSQL has shifted the paradigms in the database domain: Teams endeavoring to craft a “new database kernel” now face a formidable trial — how to stand out against the open-source, feature-rich Postgres. What’s their unique value proposition?
Until a revolutionary hardware breakthrough occurs, the advent of practical, new, general-purpose database kernels seems unlikely. No singular database can match the overall prowess of PG, bolstered by all its extensions — not even Oracle, given PG’s ace of being open-source and free.
A niche database product might carve out a space for itself if it can outperform PostgreSQL by an order of magnitude in specific aspects (typically performance). However, it usually doesn’t take long before the PostgreSQL ecosystem spawns open-source extension alternatives. Opting to develop a PG extension rather than a whole new database gives teams a crushing speed advantage in playing catch-up!
Following this logic, the PostgreSQL ecosystem is poised to snowball, accruing advantages and inevitably moving towards a monopoly, mirroring the Linux kernel’s status in server OS within a few years. Developer surveys and database trend reports confirm this trajectory.
PostgreSQL has long been the favorite database in HackerNews & StackOverflow. Many new open-source projects default to PostgreSQL as their primary, if not only, database choice. And many new-gen companies are going All in PostgreSQL.
As “Radical Simplicity: Just Use Postgres” says, Simplifying tech stacks, reducing components, accelerating development, lowering risks, and adding more features can be achieved by “Just Use Postgres.” Postgres can replace many backend technologies, including MySQL, Kafka, RabbitMQ, ElasticSearch, Mongo, and Redis, effortlessly serving millions of users. Just Use Postgres is no longer limited to a few elite teams but becoming a mainstream best practice.
What Else Can Be Done?
The endgame for the database domain seems predictable. But what can we do, and what should we do?
PostgreSQL is already a near-perfect database kernel for the vast majority of scenarios, making the idea of a kernel “bottleneck” absurd. Forks of PostgreSQL and MySQL that tout kernel modifications as selling points are essentially going nowhere.
This is similar to the situation with the Linux OS kernel today; despite the plethora of Linux distros, everyone opts for the same kernel. Forking the Linux kernel is seen as creating unnecessary difficulties, and the industry frowns upon it.
Accordingly, the main conflict is no longer the database kernel itself but two directions— database extensions and services! The former pertains to internal extensibility, while the latter relates to external composability. Much like the OS ecosystem, the competitive landscape will concentrate on database distributions. In the database domain, only those distributions centered around extensions and services stand a chance for ultimate success.
Kernel remains lukewarm, with MariaDB, the fork of MySQL’s parent, nearing delisting, while AWS, profiting from offering services and extensions on top of the free kernel, thrives. Investment has flowed into numerous PG ecosystem extensions and service distributions: Citus, TimescaleDB, Hydra, PostgresML, ParadeDB, FerretDB, StackGres, Aiven, Neon, Supabase, Tembo, PostgresAI, and our own PG distro — — Pigsty.
A dilemma within the PostgreSQL ecosystem is the independent evolution of many extensions and tools, lacking a unifier to synergize them. For instance, Hydra releases its own package and Docker image, and so does PostgresML, each distributing PostgreSQL images with their own extensions and only their own. These images and packages are far from comprehensive database services like AWS RDS.
Even service providers and ecosystem integrators like AWS fall short in front of numerous extensions, unable to include many due to various reasons (AGPLv3 license, security challenges with multi-tenancy), thus failing to leverage the synergistic amplification potential of PostgreSQL ecosystem extensions.
Extesion Category Pigsty RDS & PGDG AWS RDS PG Aliyun RDS PG Add Extension Free to Install Not Allowed Not Allowed Geo Spatial PostGIS 3.4.2 PostGIS 3.4.1 PostGIS 3.3.4 Time Series TimescaleDB 2.14.2 Distributive Citus 12.1 AI / ML PostgresML 2.8.1 Columnar Hydra 1.1.1 Vector PGVector 0.6 PGVector 0.6 pase 0.0.1 Sparse Vector PG Sparse 0.5.6 Full-Text Search pg_bm25 0.5.6 Graph Apache AGE 1.5.0 GraphQL PG GraphQL 1.5.0 Message Queue pgq 3.5.0 OLAP pg_analytics 0.5.6 DuckDB duckdb_fdw 1.1 CDC wal2json 2.5.3 wal2json 2.5 Bloat Control pg_repack 1.5.0 pg_repack 1.5.0 pg_repack 1.4.8 Point Cloud PG PointCloud 1.2.5 Ganos PointCloud 6.1 Many important extensions are not available on Cloud RDS (PG 16, 2024-02-29)
Extensions are the soul of PostgreSQL. A Postgres without the freedom to use extensions is like cooking without salt, a giant constrained.
Addressing this issue is one of our primary goals.
Our Resolution: Pigsty
Despite earlier exposure to MySQL Oracle, and MSSQL, when I first used PostgreSQL in 2015, I was convinced of its future dominance in the database realm. Nearly a decade later, I’ve transitioned from a user and administrator to a contributor and developer, witnessing PG’s march toward that goal.
Interactions with diverse users revealed that the database field’s shortcoming isn’t the kernel anymore — PostgreSQL is already sufficient. The real issue is leveraging the kernel’s capabilities, which is the reason behind RDS’s booming success.
However, I believe this capability should be as accessible as free software, like the PostgreSQL kernel itself — available to every user, not just renting from cyber feudal lords.
Thus, I created Pigsty, a battery-included, local-first PostgreSQL distribution as an open-source RDS Alternative, which aims to harness the collective power of PostgreSQL ecosystem extensions and democratize access to production-grade database services.
Pigsty stands for PostgreSQL in Great STYle, representing the zenith of PostgreSQL.
We’ve defined six core propositions addressing the central issues in PostgreSQL database services:
Extensible Postgres, Reliable Infras, Observable Graphics, Available Services, Maintainable Toolbox, and Composable Modules.
The initials of these value propositions offer another acronym for Pigsty:
Postgres, Infras, Graphics, Service, Toolbox, Yours.
Your graphical Postgres infrastructure service toolbox.
Extensible PostgreSQL is the linchpin of this distribution. In the recently launched Pigsty v2.6, we integrated DuckDB FDW and ParadeDB extensions, massively boosting PostgreSQL’s analytical capabilities and ensuring every user can easily harness this power.
Our aim is to integrate the strengths within the PostgreSQL ecosystem, creating a synergistic force akin to the Ubuntu of the database world. I believe the kernel debate is settled, and the real competitive frontier lies here.
- PostGIS: Provides geospatial data types and indexes, the de facto standard for GIS (& pgPointCloud, pgRouting).
- TimescaleDB: Adds time-series, continuous aggregates, distributed, columnar storage, and automatic compression capabilities.
- PGVector: Support AI vectors/embeddings and ivfflat, hnsw vector indexes (& pg_sparse for sparse vectors).
- Citus: Transforms classic master-slave PG clusters into horizontally partitioned distributed database clusters.
- Hydra: Adds columnar storage and analytics, rivaling ClickHouse’s analytic capabilities.
- ParadeDB: Elevates full-text search and mixed retrieval to ElasticSearch levels (& zhparser for Chinese tokenization).
- Apache AGE: Graph database extension, adding Neo4J-like OpenCypher query support to PostgreSQL.
- PG GraphQL: Adds native built-in GraphQL query language support to PostgreSQL.
- DuckDB FDW: Enables direct access to DuckDB’s powerful embedded analytic database files through PostgreSQL (& DuckDB CLI).
- Supabase: An open-source Firebase alternative based on PostgreSQL, providing a complete app development storage solution.
- FerretDB: An open-source MongoDB alternative based on PostgreSQL, compatible with MongoDB APIs/drivers.
- PostgresML: Facilitates classic machine learning algorithms, calling, deploying, and training AI models with SQL.
Developers, your choices will shape the future of the database world. I hope my work helps you better utilize the world’s most advanced open-source database kernel: PostgreSQL.
Read in Pigsty’s Blog | GitHub Repo: Pigsty | Official Website
PostgreSQL Convention 2024
- Background
- 0x01 Naming Convention
- 0x01 Design Convention
- 0x01 Query Convention
- 0x01 Admin Convention
Roughly translated from PostgreSQL Convention 2024 with Google.
0x00 Background
No Rules, No Lines
The functions of PostgreSQL are very powerful, but to use PostgreSQL well requires the cooperation of backend, operation and maintenance, and DBA.
This article has compiled a development/operation and maintenance protocol based on the principles and characteristics of the PostgreSQL database, hoping to reduce the confusion you encounter when using the PostgreSQL database: hello, me, everyone.
The first version of this article is mainly for PostgreSQL 9.4 - PostgreSQL 10. The latest version has been updated and adjusted for PostgreSQL 15/16.
0x01 naming convention
There are only two hard problems in computer science: cache invalidation and naming .
Generic naming rules (Generic)
- This rule applies to all objects in the database , including: library names, table names, index names, column names, function names, view names, serial number names, aliases, etc.
- The object name must use only lowercase letters, underscores, and numbers, and the first letter must be a lowercase letter.
- The length of the object name must not exceed 63 characters, and the naming
snake_case
style must be uniform. - The use of SQL reserved words is prohibited, use
select pg_get_keywords();
to obtain a list of reserved keywords. - Dollar signs are prohibited
$
, Chinese characters are prohibited, and do notpg
begin with . - Improve your wording taste and be honest and elegant; do not use pinyin, do not use uncommon words, and do not use niche abbreviations.
Cluster naming rules (Cluster)
- The name of the PostgreSQL cluster will be used as the namespace of the cluster resource and must be a valid DNS domain name without any dots or underscores.
- The cluster name should start with a lowercase letter, contain only lowercase letters, numbers, and minus signs, and conform to the regular expression:
[a-z][a-z0-9-]*
. - PostgreSQL database cluster naming usually follows a three-part structure:
pg-<biz>-<tld>
. Database type/business name/business line or environment biz
The English words that best represent the characteristics of the business should only consist of lowercase letters and numbers, and should not contain hyphens-
.- When using a backup cluster to build a delayed slave database of an existing cluster,
biz
the name should be<biz>delay
, for examplepg-testdelay
. - When branching an existing cluster, you can
biz
add a number at the end of : for example,pg-user1
you can branch frompg-user2
,pg-user3
etc. - For horizontally sharded clusters,
biz
the name should includeshard
and be preceded by the shard number, for examplepg-testshard1
,pg-testshard2
,… <tld>
It is the top-level business line and can also be used to distinguish different environments: for example-tt
,-dev
,-uat
,-prod
etc. It can be omitted if not required.
Service naming rules (Service)
- Each PostgreSQL cluster will provide 2 to 6 types of external services, which use fixed naming rules by default.
- The service name is prefixed with the cluster name and the service type is suffixed, for example
pg-test-primary
,pg-test-replica
. - Read-write services are uniformly
primary
named with the suffix, and read-only services are uniformlyreplica
named with the suffix. These two services are required. - ETL pull/individual user query is
offline
named with the suffix, and direct connection to the main database/ETL write isdefault
named with the suffix, which is an optional service. - The synchronous read service is
standby
named with the suffix, and the delayed slave library service isdelayed
named with the suffix. A small number of core libraries can provide this service.
Instance naming rules (Instance)
- A PostgreSQL cluster consists of at least one instance, and each instance has a unique instance number assigned from zero or one within the cluster.
- The instance name
-
is composed of the cluster name + instance number with hyphens , for example:pg-test-1
,pg-test-2
. - Once assigned, the instance number cannot be modified until the instance is offline and destroyed, and cannot be reassigned for use.
- The instance name will be used as a label for monitoring system data
ins
and will be attached to all data of this instance. - If you are using a host/database 1:1 exclusive deployment, the node Hostname can use the database instance name.
Database naming rules (Database)
- The database name should be consistent with the cluster and application, and must be a highly distinguishable English word.
- The naming is
<tld>_<biz>
constructed in the form of ,<tld>
which is the top-level business line. It can also be used to distinguish different environments and can be omitted if not used. <biz>
For a specific business name, for example,pg-test-tt
the cluster can use the library namett_test
ortest
. This is not mandatory, i.e. it is allowed to create<biz>
other databases with different cluster names.- For sharded libraries,
<biz>
the section mustshard
end with but should not contain the shard number, for examplepg-testshard1
,pg-testshard2
bothtestshard
should be used. - Multiple parts use
-
joins. For example:<biz>-chat-shard
,<biz>-payment
etc., no more than three paragraphs in total.
Role naming convention (Role/User)
dbsu
There is only one database super user :postgres
, the user used for streaming replication is namedreplicator
.- The users used for monitoring are uniformly named
dbuser_monitor
, and the super users used for daily management are:dbuser_dba
. - The business user used by the program/service defaults to using
dbuser_<biz>
as the username, for exampledbuser_test
. Access from different services should be differentiated using separate business users. - The database user applied for by the individual user agrees to use
dbp_<name>
, where isname
the standard user name in LDAP. - The default permission group naming is fixed as:
dbrole_readonly
,dbrole_readwrite
,dbrole_admin
,dbrole_offline
.
Schema naming rules (Schema)
- The business uniformly uses a global
<prefix>
as the schema name, as short as possible, and is set tosearch_path
the first element by default. <prefix>
You must not usepublic
,monitor
, and must not conflict with any schema name used by PostgreSQL extensions, such as:timescaledb
,citus
,repack
,graphql
,net
,cron
,… It is not appropriate to use special names:dba
,trash
.- Sharding mode naming rules adopt:
rel_<partition_total_num>_<partition_index>
. The middle is the total number of shards, which is currently fixed at 8192. The suffix is the shard number, counting from 0. Such asrel_8192_0
,…,,,rel_8192_11
etc. - Creating additional schemas, or using
<prefix>
schema names other than , will require R&D to explain their necessity.
Relationship naming rules (Relation)
- The first priority for relationship naming is to have clear meaning. Do not use ambiguous abbreviations or be too lengthy. Follow general naming rules.
- Table names should use plural nouns and be consistent with historical conventions. Words with irregular plural forms should be avoided as much as possible.
- Views use
v_
as the naming prefix, materialized views usemv_
as the naming prefix, temporary tables usetmp_
as the naming prefix. - Inherited or partitioned tables should be prefixed by the parent table name and suffixed by the child table attributes (rules, shard ranges, etc.).
- The time range partition uses the starting interval as the naming suffix. If the first partition has no upper bound, the R&D will specify a far enough time point: grade partition:
tbl_2023
, month-level partitiontbl_202304
, day-level partitiontbl_20230405
, hour-level partitiontbl_2023040518
. The default partition_default
ends with . - The hash partition is named with the remainder as the suffix of the partition table name, and the list partition is manually specified by the R&D team with a reasonable partition table name corresponding to the list item.
Index naming rules (Index)
- When creating an index, the index name should be specified explicitly and consistent with the PostgreSQL default naming rules.
- Index names are prefixed with the table name, primary key indexes
_pkey
end with , unique indexes_key
end with , ordinary indexes end_idx
with , and indexes used forEXCLUDED
constraints_excl
end with . - When using conditional index/function index, the function and condition content used should be reflected in the index name. For example
tbl_md5_title_idx
,tbl_ts_ge_2023_idx
, but the length limit cannot be exceeded.
Field naming rules (Attribute)
- It is prohibited to use system column reserved field names:
oid
,xmin
,xmax
,cmin
,cmax
,ctid
. - Primary key columns are usually named with
id
or asid
a suffix. - The conventional name is the creation time field
created_time
, and the conventional name is the last modification time field.updated_time
is_
It is recommended to use , etc. as the prefix for Boolean fieldshas_
.- Additional flexible JSONB fields are fixed using
extra
as column names. - The remaining field names must be consistent with existing table naming conventions, and any field naming that breaks conventions should be accompanied by written design instructions and explanations.
Enumeration item naming (Enum)
- Enumeration items should be used by default
camelCase
, but other styles are allowed.
Function naming rules (Function)
- Function names start with verbs:
select
,insert
,delete
,update
,upsert
,create
,…. - Important parameters can be reflected in the function name through
_by_ids
the_by_user_ids
suffix of. - Avoid function overloading and try to keep only one function with the same name.
BIGINT/INTEGER/SMALLINT
It is forbidden to overload function signatures through integer types such as , which may cause ambiguity when calling.- Use named parameters for variables in stored procedures and functions, and avoid positional parameters (
$1
,$2
,…). - If the parameter name conflicts with the object name, add before the parameter
_
, for example_user_id
.
Comment specifications (Comment)
- Try your best to provide comments (
COMMENT
) for various objects. Comments should be in English, concise and concise, and one line should be used. - When the object’s schema or content semantics change, be sure to update the annotations to keep them in sync with the actual situation.
0x02 Design Convention
To each his own
Things to note when creating a table
- The DDL statement for creating a table needs to use the standard format, with SQL keywords in uppercase letters and other words in lowercase letters.
- Use lowercase letters uniformly in field names/table names/aliases, and try not to be case-sensitive. If you encounter a mixed case, or a name that conflicts with SQL keywords, you need to use double quotation marks for quoting.
- Use specialized type (NUMERIC, ENUM, INET, MONEY, JSON, UUID, …) if applicable, and avoid using
TEXT
type as much as possible. TheTEXT
type is not conducive to the database’s understanding of the data. Use these types to improve data storage, query, indexing, and calculation efficiency, and improve maintainability. - Optimizing column layout and alignment types can have additional performance/storage gains.
- Unique constraints must be guaranteed by the database, and any unique column must have a corresponding unique constraint.
EXCLUDE
Constraints are generalized unique constraints that can be used to ensure data integrity in low-frequency update scenarios.
Partition table considerations
- If a single table exceeds hundreds of TB, or the monthly incremental data exceeds more than ten GB, you can consider table partitioning.
- A guideline for partitioning is to keep the size of each partition within the comfortable range of 1GB to 64GB.
- Tables that are conditionally partitioned by time range are first partitioned by time range. Commonly used granularities include: decade, year, month, day, and hour. The partitions required in the future should be created at least three months in advance.
- For extremely skewed data distributions, different time granularities can be combined, for example: 1900 - 2000 as one large partition, 2000 - 2020 as year partitions, and after 2020 as month partitions. When using time partitioning, the table name uses the value of the lower limit of the partition (if infinity, use a value that is far enough back).
Notes on wide tables
- Wide tables (for example, tables with dozens of fields) can be considered for vertical splitting, with mutual references to the main table through the same primary key.
- Because of the PostgreSQL MVCC mechanism, the write amplification phenomenon of wide tables is more obvious, reducing frequent updates to wide tables.
- In Internet scenarios, it is allowed to appropriately lower the normalization level and reduce multi-table connections to improve performance.
Primary key considerations
- Every table must have an identity column , and in principle it must have a primary key. The minimum requirement is to have a non-null unique constraint .
- The identity column is used to uniquely identify any tuple in the table, and logical replication and many third-party tools depend on it.
- If the primary key contains multiple columns, it should be specified using a single column after creating the field list of the table DDL
PRIMARY KEY(a,b,...)
. - In principle, it is recommended to use integer
UUID
types for primary keys, which can be used with caution and text types with limited length. Using other types requires explicit explanation and evaluation. - The primary key usually uses a single integer column. In principle, it is recommended to use it
BIGINT
. Use it with cautionINTEGER
and it is not allowedSMALLINT
. - The primary key should be used to
GENERATED ALWAYS AS IDENTITY
generate a unique primary key;SERIAL
,BIGSERIAL
which is only allowed when compatibility with PG versions below 10 is required. - The primary key can use
UUID
the type as the primary key, and it is recommended to use UUID v1/v7; use UUIDv4 as the primary key with caution, as random UUID has poor locality and has a collision probability. - When using a string column as a primary key, you should add a length limit. Generally used
VARCHAR(64)
, use of longer strings should be explained and evaluated. INSERT/UPDATE
In principle, it is forbidden to modify the value of the primary key column, andINSERT RETURNING
it can be used to return the automatically generated primary key value.
Foreign key considerations
- When defining a foreign key, the reference must explicitly set the corresponding action:
SET NULL
,SET DEFAULT
,CASCADE
, and use cascading operations with caution. - The columns referenced by foreign keys need to be primary key columns in other tables/this table.
- Internet businesses, especially partition tables and horizontal shard libraries, use foreign keys with caution and can be solved at the application layer.
Null/Default Value Considerations
- If there is no distinction between zero and null values in the field semantics, null values are not allowed and
NOT NULL
constraints must be configured for the column. - If a field has a default value semantically,
DEFAULT
the default value should be configured.
Numeric type considerations
- Used for regular numeric fields
INTEGER
. Used for numeric columns whose capacity is uncertainBIGINT
. - Don’t use it without special reasons
SMALLINT
. The performance and storage improvements are very small, but there will be many additional problems. - Note that the SQL standard does not provide unsigned integers, and values exceeding
INTMAX
but not exceedingUINTMAX
need to be upgraded and stored. Do not store moreINT64MAX
values inBIGINT
the column as it will overflow into negative numbers. REAL
Represents a 4-byte floating point number,FLOAT
represents an 8-byte floating point number. Floating point numbers can only be used in scenarios where the final precision doesn’t matter, such as geographic coordinates. Remember not to use equality judgment on floating point numbers, except for zero values .- Use exact numeric types
NUMERIC
. If possible, useNUMERIC(p)
andNUMERIC(p,s)
to set the number of significant digits and the number of significant digits in the decimal part. For example, the temperature in Celsius (37.0
) canNUMERIC(3,1)
be stored with 3 significant digits and 1 decimal place using type. - Currency value type is used
MONEY
.
Text type considerations
- PostgreSQL text types include
char(n)
,varchar(n)
,text
. By default,text
the type can be used, which does not limit the string length, but is limited by the maximum field length of 1GB. - If conditions permit, it is preferable to use
varchar(n)
the type to set a maximum string length. This will introduce minimal additional checking overhead, but can avoid some dirty data and corner cases. - Avoid use
char(n)
, this type has unintuitive behavior (padding spaces and truncation) and has no storage or performance advantages in order to be compatible with the SQL standard.
Time type considerations
- There are only two ways to store time: with time zone
TIMESTAMPTZ
and without time zoneTIMESTAMP
. - It is recommended to use one with time zone
TIMESTAMPTZ
. If you useTIMESTAMP
storage, you must use 0 time zone standard time. - Please use it to generate 0 time zone time
now() AT TIME ZONE 'UTC'
. You cannot truncate the time zone directlynow()::TIMESTAMP
. - Uniformly use ISO-8601 format input and output time type:
2006-01-02 15:04:05
to avoid DMY and MDY problems. - Users in China can use
Asia/Hong_Kong
the +8 time zone uniformly because the Shanghai time zone abbreviationCST
is ambiguous.
Notes on enumeration types
- Fields that are more stable and have a small value space (within tens to hundreds) should use enumeration types instead of integers and strings.
- Enumerations are internally implemented using dynamic integers, which have readability advantages over integers and performance, storage, and maintainability advantages over strings.
- Enumeration items can only be added, not deleted, but existing enumeration values can be renamed.
ALTER TYPE <enum_name>
Used to modify enumerations.
UUID type considerations
- Please note that the fully random UUIDv4 has poor locality when used as a primary key. Consider using UUIDv1/v7 instead if possible.
- Some UUID generation/processing functions require additional extension plug-ins, such as
uuid-ossp
,pg_uuidv7
etc. If you have this requirement, please specify it during configuration.
JSON type considerations
- Unless there is a special reason, always use the binary storage
JSONB
type and related functions instead of the text versionJSON
. - Note the subtle differences between atomic types in JSON and their PostgreSQL counterparts: the zero character
text
is not allowed in the type corresponding to a JSON string\u0000
, and the andnumeric
is not allowed in the type corresponding to a JSON numeric type . Boolean values only accept lowercase and literal values.NaN``infinity``true``false
- Please note that objects in the JSON standard
null
and null values in the SQL standardNULL
are not the same concept.
Array type considerations
- When storing a small number of elements, array fields can be used instead of individually.
- Suitable for storing data with a relatively small number of elements and infrequent changes. If the number of elements in the array is very large or changes frequently, consider using a separate table to store the data and using foreign key associations.
- For high-dimensional floating-point arrays, consider using
pgvector
the dedicated data types provided by the extension.
GIS type considerations
- The GIS type uses the srid=4326 reference coordinate system by default.
- Longitude and latitude coordinate points should use the Geography type without explicitly specifying the reference system coordinates 4326
Trigger considerations
- Triggers will increase the complexity and maintenance cost of the database system, and their use is discouraged in principle. The use of rule systems is prohibited and such requirements should be replaced by triggers.
- Typical scenarios for triggers are to automatically modify a row to the current timestamp after modifying it
updated_time
, or to record additions, deletions, and modifications of a table to another log table, or to maintain business consistency between the two tables. - Operations in triggers are transactional, meaning if the trigger or operations in the trigger fail, the entire transaction is rolled back, so test and prove the correctness of your triggers thoroughly. Special attention needs to be paid to recursive calls, deadlocks in complex query execution, and the execution sequence of multiple triggers.
Stored procedure/function considerations
-
Functions/stored procedures are suitable for encapsulating transactions, reducing concurrency conflicts, reducing network round-trips, reducing the amount of returned data, and executing a small amount of custom logic.
-
Stored procedures are not suitable for complex calculations, and are not suitable for trivial/frequent type conversion and packaging. In critical high-load systems, unnecessary computationally intensive logic in the database should be removed, such as using SQL in the database to convert WGS84 to other coordinate systems. Calculation logic closely related to data acquisition and filtering can use functions/stored procedures: for example, geometric relationship judgment in PostGIS.
-
Replaced functions and stored procedures that are no longer in use should be taken offline in a timely manner to avoid conflicts with future functions.
-
Use a unified syntax format for function creation. The signature occupies a separate line (function name and parameters), the return value starts on a separate line, and the language is the first label. Be sure to mark the function volatility level:
IMMUTABLE
,STABLE
,VOLATILE
. Add attribute tags, such as:RETURNS NULL ON NULL INPUT
,PARALLEL SAFE
,ROWS 1
etc.CREATE OR REPLACE FUNCTION nspname.myfunc(arg1_ TEXT, arg2_ INTEGER) RETURNS VOID LANGUAGE SQL STABLE PARALLEL SAFE ROWS 1 RETURNS NULL ON NULL INPUT AS $function$ SELECT 1; $function$;
Use sensible Locale options
- Used by default
en_US.UTF8
and cannot be changed without special reasons. - The default
collate
rule must beC
, to avoid string indexing problems. - https://mp.weixin.qq.com/s/SEXcyRFmdXNI7rpPUB3Zew
Use reasonable character encoding and localization configuration
- Character encoding must be used
UTF8
, any other character encoding is strictly prohibited. - Must be used
C
asLC_COLLATE
the default collation, any special requirements must be explicitly specified in the DDL/query clause to implement. - Character set
LC_CTYPE
is used by defaulten_US.UTF8
, some extensions rely on character set information to work properly, such aspg_trgm
.
Notes on indexing
- All online queries must design corresponding indexes according to their access patterns, and full table scans are not allowed except for very small tables.
- Indexes have a price, and it is not allowed to create unused indexes. Indexes that are no longer used should be cleaned up in time.
- When building a joint index, columns with high differentiation and selectivity should be placed first, such as ID, timestamp, etc.
- GiST index can be used to solve the nearest neighbor query problem, and traditional B-tree index cannot provide good support for KNN problem.
- For data whose values are linearly related to the storage order of the heap table, if the usual query is a range query, it is recommended to use the BRIN index. The most typical scenario is to only append written time series data. BRIN index is more efficient than Btree.
- When retrieving against JSONB/array fields, you can use GIN indexes to speed up queries.
Clarify the order of null values in B-tree indexes
NULLS FIRST
If there is a sorting requirement on a nullable column, it needs to be explicitly specified in the query and indexNULLS LAST
.- Note that
DESC
the default rule for sorting isNULLS FIRST
that null values appear first in the sort, which is generally not desired behavior. - The sorting conditions of the index must match the query, such as:
CREATE INDEX ON tbl (id DESC NULLS LAST);
Disable indexing on large fields
- The size of the indexed field cannot exceed 2KB (1/3 of the page capacity). You need to be careful when creating indexes on text types. The text to be indexed should use
varchar(n)
types with length constraints. - When a text type is used as a primary key, a maximum length must be set. In principle, the length should not exceed 64 characters. In special cases, the evaluation needs to be explicitly stated.
- If there is a need for large field indexing, you can consider hashing the large field and establishing a function index. Or use another type of index (GIN).
Make the most of functional indexes
- Any redundant fields that can be inferred from other fields in the same row can be replaced using functional indexes.
- For statements that often use expressions as query conditions, you can use expression or function indexes to speed up queries.
- Typical scenario: Establish a hash function index on a large field, and establish a
reverse
function index for text columns that require left fuzzy query.
Take advantage of partial indexes
- For the part of the query where the query conditions are fixed, partial indexes can be used to reduce the index size and improve query efficiency.
- If a field to be indexed in a query has only a limited number of values, several corresponding partial indexes can also be established.
- If the columns in some indexes are frequently updated, please pay attention to the expansion of these indexes.
0x03 Query Convention
The limits of my language mean the limits of my world.
—Ludwig Wittgenstein
Use service access
- Access to the production database must be through domain name access services , and direct connection using IP addresses is strictly prohibited.
- VIP is used for services and access, LVS/HAProxy shields the role changes of cluster instance members, and master-slave switching does not require application restart.
Read and write separation
- Internet business scenario: Write requests must go through the main library and be accessed through the Primary service.
- In principle, read requests go from the slave library and are accessed through the Replica service.
- Exceptions: If you need “Read Your Write” consistency guarantees, and significant replication delays are detected, read requests can access the main library; or apply to the DBA to provide Standby services.
Separation of speed and slowness
- Queries within 1 millisecond in production are called fast queries, and queries that exceed 1 second in production are called slow queries.
- Slow queries must go to the offline slave database - Offline service/instance, and a timeout should be set during execution.
- In principle, the execution time of online general queries in production should be controlled within 1ms.
- If the execution time of an online general query in production exceeds 10ms, the technical solution needs to be modified and optimized before going online.
- Online queries should be configured with a Timeout of the order of 10ms or faster to avoid avalanches caused by accumulation.
- ETL data from the primary is prohibited, and the offline service should be used to retrieve data from a dedicated instance.
Use connection pool
- Production applications must access the database through a connection pool and the PostgreSQL database through a 1:1 deployed Pgbouncer proxy. Offline service, individual users are strictly prohibited from using the connection pool directly.
- Pgbouncer connection pool uses Transaction Pooling mode by default. Some session-level functions may not be available (such as Notify/Listen), so special attention is required. Pre-1.21 Pgbouncer does not support the use of Prepared Statements in this mode. In special scenarios, you can use Session Pooling or bypass the connection pool to directly access the database, which requires special DBA review and approval.
- When using a connection pool, it is prohibited to modify the connection status, including modifying connection parameters, modifying search paths, changing roles, and changing databases. The connection must be completely destroyed after modification as a last resort. Putting the changed connection back into the connection pool will lead to the spread of contamination. Use of pg_dump to dump data via Pgbouncer is strictly prohibited.
Configure active timeout for query statements
- Applications should configure active timeouts for all statements and proactively cancel requests after timeout to avoid avalanches. (Go context)
- Statements that are executed periodically must be configured with a timeout smaller than the execution period to avoid avalanches.
- HAProxy is configured with a default connection timeout of 24 hours for rolling expired long connections. Please do not run SQL that takes more than 1 day to execute on offline instances. This requirement will be specially adjusted by the DBA.
Pay attention to replication latency
- Applications must be aware of synchronization delays between masters and slaves and properly handle situations where replication delays exceed reasonable limits.
- Under normal circumstances, replication delays are on the order of 100µs/tens of KB, but in extreme cases, slave libraries may experience replication delays of minutes/hours. Applications should be aware of this phenomenon and have corresponding degradation plans - Select Read from the main library and try again later, or report an error directly.
Retry failed transactions
- Queries may be killed due to concurrency contention, administrator commands, etc. Applications need to be aware of this and retry if necessary.
- When the application reports a large number of errors in the database, it can trigger the circuit breaker to avoid an avalanche. But be careful to distinguish the type and nature of errors.
Disconnected and reconnected
- The database connection may be terminated for various reasons, and the application must have a disconnection reconnection mechanism.
- It can be used
SELECT 1
as a heartbeat packet query to detect the presence of messages on the connection and keep it alive periodically.
Online service application code prohibits execution of DDL
- It is strictly forbidden to execute DDL in production applications and do not make big news in the application code.
- Exception scenario: Creating new time partitions for partitioned tables can be carefully managed by the application.
- Special exception: Databases used by office systems, such as Gitlab/Jira/Confluence, etc., can grant application DDL permissions.
SELECT statement explicitly specifies column names
- Avoid using it
SELECT *
, orRETURNING
use it in a clause*
. Please use a specific field list and do not return unused fields. When the table structure changes (for example, a new value column), queries that use column wildcards are likely to encounter column mismatch errors. - After the fields of some tables are maintained, the order will change. For example: after
id
upgrading the INTEGER primary key toBIGINT
,id
the column order will be the last column. This problem can only be fixed during maintenance and migration. R&D developers should resist the compulsion to adjust the column order and explicitly specify the column order in the SELECT statement. - Exception: Wildcards are allowed when a stored procedure returns a specific table row type.
Disable online query full table scan
- Exceptions: constant minimal table, extremely low-frequency operations, table/return result set is very small (within 100 records/100 KB).
- Using negative operators such as on the first-level filter condition will result in a full table scan and must be
!=
avoided .<>
Disallow long waits in transactions
- Transactions must be committed or rolled back as soon as possible after being started. Transactions that exceed 10 minutes
IDEL IN Transaction
will be forcibly killed. - Applications should enable AutoCommit to avoid
BEGIN
unpairedROLLBACK
or unpaired applications laterCOMMIT
. - Try to use the transaction infrastructure provided by the standard library, and do not control transactions manually unless absolutely necessary.
Things to note when using count
count(*)
It is the standard syntax for counting rows and has nothing to do with null values.count(col)
The count is the number of non-null recordscol
in the column . NULL values in this column will not be counted.count(distinct col)
Whencol
deduplicating columns and counting them, null values are also ignored, that is, only the number of non-null distinct values is counted.count((col1, col2))
When counting multiple columns, even if the columns to be counted are all empty, they will still be counted.(NULL,NULL)
This is valid.a(distinct (col1, col2))
For multi-column deduplication counting, even if the columns to be counted are all empty, they will be counted,(NULL,NULL)
which is effective.
Things to note when using aggregate functions
- All
count
aggregate functions exceptNULL
Butcount(col)
in this case it will be returned0
as an exception. - If returning null from an aggregate function is not expected, use
coalesce
to set a default value.
Handle null values with caution
-
Clearly distinguish between zero values and null values. Use null values
IS NULL
for equivalence judgment, and use regular=
operators for zero values for equivalence judgment. -
When a null value is used as a function input parameter, it should have a type modifier, otherwise the overloaded function will not be able to identify which one to use.
-
Pay attention to the null value comparison logic: the result of any comparison operation involving null values is
unknown
you need to pay attention tonull
the logic involved in Boolean operations:and
:TRUE or NULL
Will return due to logical short circuitTRUE
.or
:FALSE and NULL
Will return due to logical short circuitFALSE
- In other cases, as long as the operand appears
NULL
, the result isNULL
-
The result of logical judgment between null value and any value is null value, for example,
NULL=NULL
the return result isNULL
notTRUE/FALSE
. -
For equality comparisons involving null values and non-null values, please use ``IS DISTINCT FROM
-
NULL values and aggregate functions: When all input values are NULL, the aggregate function returns NULL.
Note that the serial number is empty
- When using
Serial
types,INSERT
,UPSERT
and other operations will consume sequence numbers, and this consumption will not be rolled back when the transaction fails. - When using an integer
INTEGER
as the primary key and the table has frequent insertion conflicts, you need to pay attention to the problem of integer overflow.
The cursor must be closed promptly after use
Repeated queries using prepared statements
- Prepared Statements should be used for repeated queries to eliminate the CPU overhead of database hard parsing. Pgbouncer versions earlier than 1.21 cannot support this feature in transaction pooling mode, please pay special attention.
- Prepared statements will modify the connection status. Please pay attention to the impact of the connection pool on prepared statements.
Choose the appropriate transaction isolation level
- The default isolation level is read committed , which is suitable for most simple read and write transactions. For ordinary transactions, choose the lowest isolation level that meets the requirements.
- For write transactions that require transaction-level consistent snapshots, use the Repeatable Read isolation level.
- For write transactions that have strict requirements on correctness (such as money-related), use the serializable isolation level.
- When a concurrency conflict occurs between the RR and SR isolation levels, the application should actively retry depending on the error type.
rh 09 Do not use count when judging the existence of a result.
- It is faster than Count to
SELECT 1 FROM tbl WHERE xxx LIMIT 1
judge whether there are columns that meet the conditions. SELECT exists(SELECT * FROM tbl WHERE xxx LIMIT 1)
The existence result can be converted to a Boolean value using .
Use the RETURNING clause to retrieve the modified results in one go
RETURNING
The clause can be used after theINSERT
,UPDATE
,DELETE
statement to effectively reduce the number of database interactions.
Use UPSERT to simplify logic
- When the business has an insert-failure-update sequence of operations, consider using
UPSERT
substitution.
Use advisory locks to deal with hotspot concurrency .
- For extremely high-frequency concurrent writes (spike) of single-row records, advisory locks should be used to lock the record ID.
- If high concurrency contention can be resolved at the application level, don’t do it at the database level.
Optimize IN operator
- Use
EXISTS
clause instead ofIN
operator for better performance. - Use
=ANY(ARRAY[1,2,3,4])
insteadIN (1,2,3,4)
for better results. - Control the size of the parameter list. In principle, it should not exceed 10,000. If it exceeds, you can consider batch processing.
It is not recommended to use left fuzzy search
- Left fuzzy search
WHERE col LIKE '%xxx'
cannot make full use of B-tree index. If necessary,reverse
expression function index can be used.
Use arrays instead of temporary tables
- Consider using an array instead of a temporary table, for example when obtaining corresponding records for a series of IDs.
=ANY(ARRAY[1,2,3])
Better than temporary table JOIN.
0x04 Administration Convention
Use Pigsty to build PostgreSQL cluster and infrastructure
- The production environment uses the Pigsty trunk version uniformly, and deploys the database on x86_64 machines and CentOS 7.9 / RockyLinux 8.8 operating systems.
pigsty.yml
Configuration files usually contain highly sensitive and important confidential information. Git should be used for version management and access permissions should be strictly controlled.files/pki
The CA private key and other certificates generated within the system should be properly kept, regularly backed up to a secure area for storage and archiving, and access permissions should be strictly controlled.- All passwords are not allowed to use default values, and make sure they have been changed to new passwords with sufficient strength.
- Strictly control access rights to management nodes and configuration code warehouses, and only allow DBA login and access.
Monitoring system is a must
- Any deployment must have a monitoring system, and the production environment uses at least two sets of Infra nodes to provide redundancy.
Properly plan the cluster architecture according to needs
- Any production database cluster managed by a DBA must have at least one online slave database for online failover.
- The template is used by default
oltp
, the analytical database usesolap
the template, the financial database usescrit
the template, and the micro virtual machine (within four cores) usestiny
the template. - For businesses whose annual data volume exceeds 1TB, or for clusters whose write TPS exceeds 30,000 to 50,000, you can consider building a horizontal sharding cluster.
Configure cluster high availability using Patroni and Etcd
- The production database cluster uses Patroni as the high-availability component and etcd as the DCS.
etcd
Use a dedicated virtual machine cluster, with 3 to 5 nodes, strictly scattered and distributed on different cabinets.- Patroni Failsafe mode must be turned on to ensure that the cluster main library can continue to work when etcd fails.
Configure cluster PITR using pgBackRest and MinIO
- The production database cluster uses pgBackRest as the backup recovery/PITR solution and MinIO as the backup storage warehouse.
- MinIO uses a multi-node multi-disk cluster, and can also use S3/OSS/COS services instead. Password encryption must be set for cold backup.
- All database clusters perform a local full backup every day, retain the backup and WAL of the last week, and save a full backup every other month.
- When a WAL archiving error occurs, you should check the backup warehouse and troubleshoot the problem in time.
Core business database configuration considerations
- The core business cluster needs to configure at least two online slave libraries, one of which is a dedicated offline query instance.
- The core business cluster needs to build a delayed slave cluster with a 24-hour delay for emergency data recovery.
- Core business clusters usually use asynchronous submission, while those related to money use synchronous submission.
Financial database configuration considerations
- The financial database cluster requires at least two online slave databases, one of which is a dedicated synchronization Standby instance, and Standby service access is enabled.
- Money-related libraries must use
crit
templates with RPO = 0, enable synchronous submission to ensure zero data loss, and enable Watchdog as appropriate. - Money-related libraries must be forced to turn on data checksums and, if appropriate, turn on full DML logs.
Use reasonable character encoding and localization configuration
- Character encoding must be used
UTF8
, any other character encoding is strictly prohibited. - Must be used
C
asLC_COLLATE
the default collation, any special requirements must be explicitly specified in the DDL/query clause to implement. - Character set
LC_CTYPE
is used by defaulten_US.UTF8
, some extensions rely on character set information to work properly, such aspg_trgm
.
Business database management considerations
- Multiple different databases are allowed to be created in the same cluster, and Ansible scripts must be used to create new business databases.
- All business databases must exist synchronously in the Pgbouncer connection pool.
Business user management considerations
- Different businesses/services must use different database users, and Ansible scripts must be used to create new business users.
- All production business users must be synchronized in the user list file of the Pgbouncer connection pool.
- Individual users should set a password with a default validity period of 90 days and change it regularly.
- Individual users are only allowed to access authorized cluster offline instances or slave
pg_offline_query
libraries with from the springboard machine.
Notes on extension management
yum/apt
When installing a new extension, you must first install the corresponding major version of the extension binary package in all instances of the cluster .- Before enabling the extension, you need to confirm whether the extension needs to be added
shared_preload_libraries
. If necessary, a rolling restart should be arranged. - Note that
shared_preload_libraries
in order of priority,citus
,timescaledb
,pgml
are usually placed first. pg_stat_statements
andauto_explain
are required plugins and must be enabled in all clusters.- Install extensions uniformly using , and create them
dbsu
in the business database .CREATE EXTENSION
Database XID and age considerations
- Pay attention to the age of the database and tables to avoid running out of XID transaction numbers. If the usage exceeds 20%, you should pay attention; if it exceeds 50%, you should intervene immediately.
- When processing XID, execute the table one by one in order of age from largest to smallest
VACUUM FREEZE
.
Database table and index expansion considerations
- Pay attention to the expansion rate of tables and indexes to avoid index performance degradation, and use
pg_repack
online processing to handle table/index expansion problems. - Generally speaking, indexes and tables whose expansion rate exceeds 50% can be considered for reorganization.
- When dealing with table expansion exceeding 100GB, you should pay special attention and choose business low times.
Database restart considerations
- Before restarting the database, execute it
CHECKPOINT
twice to force dirty pages to be flushed, which can speed up the restart process. - Before restarting the database, perform
pg_ctl reload
reload configuration to confirm that the configuration file is available normally. - To restart the database, use
pg_ctl restart
patronictl or patronictl to restart the entire cluster at the same time. - Use
kill -9
to shut down any database process is strictly prohibited.
Replication latency considerations
- Monitor replication latency, especially when using replication slots.
New slave database data warm-up
- When adding a new slave database instance to a high-load business cluster, the new database instance should be warmed up, and the HAProxy instance weight should be gradually adjusted and applied in gradients: 4, 8, 16, 32, 64, and 100.
pg_prewarm
Hot data can be loaded into memory using .
Database publishing process
- Online database release requires several evaluation stages: R&D self-test, supervisor review, QA review (optional), and DBA review.
- During the R&D self-test phase, R&D should ensure that changes are executed correctly in the development and pre-release environments.
- If a new table is created, the record order magnitude, daily data increment estimate, and read and write throughput magnitude estimate should be given.
- If it is a new function, the average execution time and extreme case descriptions should be given.
- If it is a mode change, all upstream and downstream dependencies must be sorted out.
- If it is a data change and record revision, a rollback SQL must be given.
- The R&D Team Leader needs to evaluate and review changes and be responsible for the content of the changes.
- The DBA evaluates and reviews the form and impact of the release, puts forward review opinions, and calls back or implements them uniformly.
Data work order format
- Database changes are made through the platform, with one work order for each change.
- The title is clear: A certain business needs
xx
to perform an action in the databaseyy
. - The goal is clear: what operations need to be performed on which instances in each step, and how to verify the results.
- Rollback plan: Any changes need to provide a rollback plan, and new ones also need to provide a cleanup script.
- Any changes need to be recorded and archived, and have complete approval records. They are first approved by the R&D superior TL Review and then approved by the DBA.
Database change release considerations
- Using a unified release window, changes of the day will be collected uniformly at 16:00 every day and executed sequentially; requirements confirmed by TL after 16:00 will be postponed to the next day. Database release is not allowed after 19:00. For emergency releases, please ask TL to make special instructions and send a copy to the CTO for approval before execution.
- Database DDL changes and DML changes are uniformly
dbuser_dba
executed remotely using the administrator user to ensure that the default permissions work properly. - When the business administrator executes DDL by himself, he must
SET ROLE dbrole_admin
first execute the release to ensure the default permissions. - Any changes require a rollback plan before they can be executed, and very few operations that cannot be rolled back need to be handled with special caution (such as enumeration of value additions)
- Database changes use
psql
command line tools, connect to the cluster main database to execute, use\i
execution scripts or\e
manual execution in batches.
Things to note when deleting tables
- The production data table
DROP
should be renamed first and allowed to cool for 1 to 3 days to ensure that it is not accessed before being removed. - When cleaning the table, you must sort out all dependencies, including directly and indirectly dependent objects: triggers, foreign key references, etc.
- The temporary table to be deleted is usually placed in
trash
Schema andALTER TABLE SET SCHEMA
the schema name is modified. - In high-load business clusters, when removing particularly large tables (> 100G), select business valleys to avoid preempting I/O.
Things to note when creating and deleting indexes
- You must use
CREATE INDEX CONCURRENTLY
concurrent index creation andDROP INDEX CONCURRENTLY
concurrent index removal. - When rebuilding an index, always create a new index first, then remove the old index, and modify the new index name to be consistent with the old index.
- After index creation fails, you should remove
INVALID
the index in time. After modifying the index, useanalyze
to re-collect statistical data on the table. - When the business is idle, you can enable parallel index creation and set it
maintenance_work_mem
to a larger value to speed up index creation.
Make schema changes carefully
- Try to avoid full table rewrite changes as much as possible. Full table rewrite is allowed for tables within 1GB. The DBA should notify all relevant business parties when the changes are made.
- When adding new columns to an existing table, you should avoid using functions in default values
VOLATILE
to avoid a full table rewrite. - When changing a column type, all functions and views that depend on that type should be rebuilt if necessary, and
ANALYZE
statistics should be refreshed.
Control the batch size of data writing
- Large batch write operations should be divided into small batches to avoid generating a large amount of WAL or occupying I/O at one time.
- After a large batch
UPDATE
is executed,VACUUM
the space occupied by dead tuples is reclaimed. - The essence of executing DDL statements is to modify the system directory, and it is also necessary to control the number of DDL statements in a batch.
Data loading considerations
- Use
COPY
load data, which can be executed in parallel if necessary. - You can temporarily shut down before loading data
autovacuum
, disable triggers as needed, and create constraints and indexes after loading. - Turn it up
maintenance_work_mem
, increase itmax_wal_size
. - Executed after loading is complete
vacuum verbose analyze table
.
Notes on database migration and major version upgrades
- The production environment uniformly uses standard migration to build script logic, and realizes requirements such as non-stop cluster migration and major version upgrades through blue-green deployment.
- For clusters that do not require downtime, you can use
pg_dump | psql
logical export and import to stop and upgrade.
Data Accidental Deletion/Accidental Update Process
- After an accident occurs, immediately assess whether it is necessary to stop the operation to stop bleeding, assess the scale of the impact, and decide on treatment methods.
- If there is a way to recover on the R&D side, priority will be given to the R&D team to make corrections through SQL publishing; otherwise, use
pageinspect
andpg_dirtyread
to rescue data from the bad table. - If there is a delayed slave library, extract data from the delayed slave library for repair. First, confirm the time point of accidental deletion, and advance the delay to extract data from the database to the XID.
- A large area was accidentally deleted and written. After communicating with the business and agreeing, perform an in-place PITR rollback to a specific time.
Data corruption processing process
- Confirm whether the slave database data can be used for recovery. If the slave database data is intact, you can switchover to the slave database first.
- Temporarily shut down
auto_vacuum
, locate the root cause of the error, replace the failed disk and add a new slave database. - If the system directory is damaged, or use to
pg_filedump
recover data from table binaries. - If the CLOG is damaged, use
dd
to generate a fake submission record.
Things to note when the database connection is full
- When the connection is full (avalanche), immediately use the kill connection query to cure the symptoms and stop the loss:
pg_cancel_backend
orpg_terminate_backend
. - Use to
pg_terminate_backend
abort all normal backend processes,psql
\watch 1
starting with once per second ( ). And confirm the connection status from the monitoring system. If the accumulation continues, continue to increase the execution frequency of the connection killing query, for example, once every 0.1 seconds until there is no more accumulation. - After confirming that the bleeding has stopped from the monitoring system, try to stop the killing connection. If the accumulation reappears, immediately resume the killing connection. Immediately analyze the root cause and perform corresponding processing (upgrade, limit current, add index, etc.)
PostgreSQL, The most successful database
The StackOverflow 2023 Survey, featuring feedback from 90K developers across 185 countries, is out. PostgreSQL topped all three survey categories (used, loved, and wanted), earning its title as the undisputed “Decathlete Database” – it’s hailed as the “Linux of Database”!
What makes a database “successful”? It’s a mix of features, quality, security, performance, and cost, but success is mainly about adoption and legacy. The size, preference, and needs of its user base are what truly shape its ecosystem’s prosperity. StackOverflow’s annual surveys for seven years have provided a window into tech trends.
PostgreSQL is now the world’s most popular database.
PostgreSQL is developers’ favorite database!
PostgreSQL sees the highest demand among users!
Popularity, the used
reflects the past, the loved
indicates the present, and the wanted
suggests the future. These metrics vividly showcase the vitality of a technology. PostgreSQL stands strong in both stock and potential, unlikely to be rivaled soon.
As a dedicated user, community member, expert, evangelist, and contributor to PostgreSQL, witnessing this moment is profoundly moving. Let’s delve into the “Why” and “What” behind this phenomenon.
Source: Community Survey
Developers define the success of databases, and StackOverflow’s survey, with popularity, love, and demand metrics, captures this directly.
“Which database environments have you done extensive development work in over the past year, and which do you want to work in over the next year? If you both worked with the database and want to continue to do so, please check both boxes in that row.”
Each database in the survey had two checkboxes: one for current use, marking the user as “Used,” and one for future interest, marking them as “Wanted.” Those who checked both were labeled as “Loved/Admired.”
The percentage of “Used” respondents represents popularity or usage rate, shown as a bar chart, while “Wanted” indicates demand or desire, marked with blue dots. “Loved/Admired” shows as red dots, indicating love or reputation. In 2023, PostgreSQL outstripped MySQL in popularity, becoming the world’s most popular database, and led by a wide margin in demand and reputation.
Reviewing seven years of data and plotting the top 10 databases on a scatter chart of popularity vs. net love percentage (2*love% - 100), we gain insights into the database field’s evolution and sense of scale.
X: Popularity, Y: Net Love Index (2 * loved - 100)
The 2023 snapshot shows PostgreSQL in the top right, popular and loved, while MySQL, popular yet less favored, sits in the bottom right. Redis, moderately popular but much loved, is in the top left, and Oracle, neither popular nor loved, is in the bottom left. In the middle lie SQLite, MongoDB, and SQL Server.
Trends indicate PostgreSQL’s growing popularity and love; MySQL’s love remains flat with falling popularity. Redis and SQLite are progressing, MongoDB is peaking and declining, and the commercial RDBMSs SQL Server and Oracle are on a downward trend.
The takeaway: PostgreSQL’s standing in the database realm, akin to Linux in server OS, seems unshakeable for the foreseeable future.
Historical Accumulation: Popularity
PostgreSQL — The world’s most popular database
Popularity is the percentage of total users who have used a technology in the past year. It reflects the accumulated usage over the past year and is a core metric of factual significance.
In 2023, PostgreSQL, branded as the “most advanced,” surpassed the “most popular” database MySQL with a usage rate of 45.6%, leading by 4.5% and reaching 1.1 times the usage rate of MySQL at 41.1%. Among professional developers (about three-quarters of the sample), PostgreSQL had already overtaken MySQL in 2022, with a 0.8 percentage point lead (46.5% vs 45.7%); this gap widened in 2023 to 49.1% vs 40.6%, or 1.2 times the usage rate among professional developers.
Over the past years, MySQL enjoyed the top spot in database popularity, proudly claiming the title of the “world’s most popular open-source relational database.” However, PostgreSQL has now claimed the crown. Compared to PostgreSQL and MySQL, other databases are not in the same league in terms of popularity.
The key trend to note is that among the top-ranked databases, only PostgreSQL has shown a consistent increase in popularity, demonstrating strong growth momentum, while all other databases have seen a decline in usage. As time progresses, the gap in popularity between PostgreSQL and other databases will likely widen, making it hard for any challenger to displace PostgreSQL in the near future.
Notably, the “domestic database” TiDB has entered the StackOverflow rankings for the first time, securing the 32nd spot with a 0.2% usage rate.
Popularity reflects the current scale and potential of a database, while love indicates its future growth potential.
Current Momentum: Love
PostgreSQL — The database developers love the most
Love or admiration is a measure of the percentage of users who are willing to continue using a technology, acting as an annual “retention rate” metric that reflects the user’s opinion and evaluation of the technology.
In 2023, PostgreSQL retained its title as the most loved database by developers. While Redis had been the favorite in previous years, PostgreSQL overtook Redis in 2022, becoming the top choice. PostgreSQL and Redis have maintained close reputation scores (around 70%), significantly outpacing other contenders.
In the 2022 PostgreSQL community survey, the majority of existing PostgreSQL users reported increased usage and deeper engagement, highlighting the stability of its core user base.
Redis, known for its simplicity and ease of use as a data structure cache server, is often paired with the relational database PostgreSQL, enjoying considerable popularity (20%, ranking sixth) among developers. Cross-analysis shows a strong connection between the two: 86% of Redis users are interested in using PostgreSQL, and 30% of PostgreSQL users want to use Redis. Other databases with positive reviews include SQLite, MongoDB, and SQL Server. MySQL and ElasticSearch receive mixed feedback, hovering around the 50% mark. The least favored databases include Access, IBM DB2, CouchDB, Couchbase, and Oracle.
Not all potential can be converted into kinetic energy. While user affection is significant, it doesn’t always translate into action, leading to the third metric of interest – demand.
Future Trends: Demand
PostgreSQL - The Most Wanted Database
The demand rate, or the level of desire, represents the percentage of users who will actually opt for a technology in the coming year. PostgreSQL stands out in demand/desire, significantly outpacing other databases with a 42.3% rate for the second consecutive year, showing relentless growth and widening the gap with its competitors.
In 2023, some databases saw notable demand increases, likely driven by the surge in large language model AI, spearheaded by OpenAI’s ChatGPT. This demand for intelligence has, in turn, fueled the need for robust data infrastructure. A decade ago, support for NoSQL features like JSONB/GIN laid the groundwork for PostgreSQL’s explosive growth during the internet boom. Today, the introduction of pgvector, the first vector extension built on a mature database, grants PostgreSQL a ticket into the AI era, setting the stage for growth in the next decade.
But Why?
PostgreSQL leads in demand, usage, and popularity, with the right mix of timing, location, and human support, making it arguably the most successful database with no visible challengers in the near future. The secret to its success lies in its slogan: “The World’s Most Advanced Open Source Relational Database.”
Relational databases are so prevalent and crucial that they might dwarf the combined significance of other types like key-value, document, search engine, time-series, graph, and vector databases. Typically, “database” implicitly refers to “relational database,” where no other category dares claim mainstream status. Last year’s “Why PostgreSQL Will Be the Most Successful Database?” delves into the competitive landscape of relational databases—a tripartite dominance. Excluding Microsoft’s relatively isolated SQL Server, the database scene, currently in a phase of consolidation, has three key players rooted in WireProtocol: Oracle, MySQL, and PostgreSQL, mirroring a “Three Kingdoms” saga in the relational database realm.
Oracle/MySQL are waning, while PostgreSQL is thriving. Oracle is an established commercial DB with deep tech history, rich features, and strong support, favored by well-funded, risk-averse enterprises, especially in finance. Yet, it’s pricey and infamous for litigious practices. MS SQL Server shares similar traits with Oracle. Commercial databases are facing a slow decline due to the open-source wave.
MySQL, popular yet beleaguered, lags in stringent transaction processing and data analysis compared to PostgreSQL. Its agile development approach is also outperformed by NoSQL alternatives. Oracle’s dominance, sibling rivalry with MariaDB, and competition from NewSQL players like TiDB/OB contribute to its decline.
Oracle, no doubt skilled, lacks integrity, hence “talented but unprincipled.” MySQL, despite its open-source merit, is limited in capability and sophistication, hence “limited talent, weak ethics.” PostgreSQL, embodying both capability and integrity, aligns with the open-source rise, popular demand, and advanced stability, epitomizing “talented and principled.”
Open Source & Advanced
The primary reasons for choosing PostgreSQL, as reflected in the TimescaleDB community survey, are its open-source nature and stability. Open-source implies free use, potential for modification, no vendor lock-in, and no “chokepoint” issues. Stability means reliable, consistent performance with a proven track record in large-scale production environments. Experienced developers value these attributes highly.
Broadly, aspects like extensibility, ecosystem, community, and protocols fall under “open-source.” Stability, ACID compliance, SQL support, scalability, and availability define “advanced.” These resonate with PostgreSQL’s slogan: “The world’s most advanced open source relational database.”
The Virtue of Open Source
powered by developers worldwide. Friendly BSD license, thriving ecosystem, extensive expansion. A robust Oracle alternative, leading the charge.
What is “virtue”? It’s the manifestation of “the way,” and this way is open source. PostgreSQL stands as a venerable giant among open-source projects, epitomizing global collaborative success.
Back in the day, developing software/information services required exorbitantly priced commercial databases. Just the software licensing fees could hit six or seven figures, not to mention similar costs for hardware and service subscriptions. Oracle’s licensing fee per CPU core could reach hundreds of thousands annually, prompting even giants like Alibaba to seek IOE alternatives. The rise of open-source databases like PostgreSQL and MySQL offered a fresh choice.
Open-source databases, free of charge, spurred an industry revolution: from tens of thousands per core per month for commercial licenses to a mere 20 bucks per core per month for hardware. Databases became accessible to regular businesses, enabling the provision of free information services.
Open source has been monumental: the history of the internet is a history of open-source software. The prosperity of the IT industry and the plethora of free information services owe much to open-source initiatives. Open source represents a form of successful Communism in software, with the industry’s core means of production becoming communal property, available to developers worldwide as needed. Developers contribute according to their abilities, embracing the ethos of mutual benefit.
An open-source programmer’s work encapsulates the intellect of countless top-tier developers. Programmers command high salaries because they are not mere laborers but contractors orchestrating software and hardware. They own the core means of production: software from the public domain and readily available server hardware. Thus, a few skilled engineers can swiftly tackle domain-specific problems leveraging the open-source ecosystem.
Open source synergizes community efforts, drastically reducing redundancy and propelling technical advancements at an astonishing pace. Its momentum, now unstoppable, continues to grow like a snowball. Open source dominates foundational software, and the industry now views insular development or so-called “self-reliance” in software, especially in foundational aspects, as a colossal joke.
For PostgreSQL, open source is its strongest asset against Oracle.
Oracle is advanced, but PostgreSQL holds its own. It’s the most Oracle-compatible open-source database, natively supporting 85% of Oracle’s features, with specialized distributions reaching 96% compatibility. However, the real game-changer is cost: PG’s open-source nature and significant cost advantage provide a substantial ecological niche. It doesn’t need to surpass Oracle in features; being “90% right at a fraction of the cost” is enough to outcompete Oracle.
PostgreSQL is like an open-source “Oracle,” the only real threat to Oracle’s dominance. As a leader in the “de-Oracle” movement, PG has spawned numerous “domestically controllable” database companies. According to CITIC, 36% of “domestic databases” are based on PG modifications or rebranding, with Huawei’s openGauss and GaussDB as prime examples. Crucially, PostgreSQL uses a BSD-Like license, permitting such adaptations — you can rebrand and sell without deceit. This open attitude is something Oracle-acquired, GPL-licensed MySQL can’t match.
The advanced in Talent
The talent of PG lies in its advancement. Specializing in multiple areas, PostgreSQL offers a full-stack, multi-model approach: “Self-managed, autonomous driving temporal-geospatial AI vector distributed document graph with full-text search, programmable hyper-converged, federated stream-batch processing in a single HTAP Serverless full-stack platform database”, covering almost all database needs with a single component.
PostgreSQL is not just a traditional OLTP “relational database” but a multi-modal database. For SMEs, a single PostgreSQL component can cover the vast majority of their data needs: OLTP, OLAP, time-series, GIS, tokenization and full-text search, JSON/XML documents, NoSQL features, graphs, vectors, and more.
Emperor of Databases — Self-managed, autonomous driving temporal-geospatial AI vector distributed document graph with full-text search, programmable hyper-converged, federated stream-batch processing in a single HTAP Serverless full-stack platform database.
The superiority of PostgreSQL is not only in its acclaimed kernel stability but also in its powerful extensibility. The plugin system transforms PostgreSQL from a single-threaded evolving database kernel to a platform with countless parallel-evolving extensions, exploring all possibilities simultaneously like quantum computing. PostgreSQL is omnipresent in every niche of data processing.
For instance, PostGIS for geospatial databases, TimescaleDB for time-series, Citus for distributed/columnar/HTAP databases, PGVector for AI vector databases, AGE for graph databases, PipelineDB for stream processing, and the ultimate trick — using Foreign Data Wrappers (FDW) for unified SQL access to all heterogeneous external databases. Thus, PG is a true full-stack database platform, far more advanced than a simple OLTP system like MySQL.
Within a significant scale, PostgreSQL can play multiple roles with a single component, greatly reducing project complexity and cost. Remember, designing for unneeded scale is futile and an example of premature optimization. If one technology can meet all needs, it’s the best choice rather than reimplementing it with multiple components.
Taking Tantan as an example, with 250 million TPS and 200 TB of unique TP data, a single PostgreSQL selection remains stable and reliable, covering a wide range of functions beyond its primary OLTP role, including caching, OLAP, batch processing, and even message queuing. However, as the user base approaches tens of millions daily active users, these additional functions will eventually need to be handled by dedicated components.
PostgreSQL’s advancement is also evident in its thriving ecosystem. Centered around the database kernel, there are specialized variants and “higher-level databases” built on it, like Greenplum, Supabase (an open-source alternative to Firebase), and the specialized graph database edgedb, among others. There are various open-source/commercial/cloud distributions integrating tools, like different RDS versions and the plug-and-play Pigsty; horizontally, there are even powerful mimetic components/versions emulating other databases without changing client drivers, like babelfish for SQL Server, FerretDB for MongoDB, and EnterpriseDB/IvorySQL for Oracle compatibility.
PostgreSQL’s advanced features are its core competitive strength against MySQL, another open-source relational database.
Advancement is PostgreSQL’s core competitive edge over MySQL.
MySQL’s slogan is “the world’s most popular open-source relational database,” characterized by being rough, fierce, and fast, catering to internet companies. These companies prioritize simplicity (mainly CRUD), data consistency and accuracy less than traditional sectors like banking, and can tolerate data inaccuracies over service downtime, unlike industries that cannot afford financial discrepancies.
However, times change, and PostgreSQL has rapidly advanced, surpassing MySQL in speed and robustness, leaving only “roughness” as MySQL’s remaining trait.
MySQL allows partial transaction commits by default, shocked
MySQL allows partial transaction commits by default, revealing a gap between “popular” and “advanced.” Popularity fades with obsolescence, while advancement gains popularity through innovation. In times of change, without advanced features, popularity is fleeting. Research shows MySQL’s pride in “popularity” cannot stand against PostgreSQL’s “advanced” superiority.
Advancement and open-source are PostgreSQL’s success secrets. While Oracle is advanced and MySQL is open-source, PostgreSQL boasts both. With the right conditions, success is inevitable.
Looking Ahead
The PostgreSQL database kernel’s role in the database ecosystem mirrors the Linux kernel’s in the operating system domain. For databases, particularly OLTP, the battle of kernels has settled—PostgreSQL is now a perfect engine.
However, users need more than an engine; they need the complete car, driving capabilities, and traffic services. The database competition has shifted from software to Software enabled Service—complete database distributions and services. The race for PostgreSQL-based distributions is just beginning. Who will be the PostgreSQL equivalent of Debian, RedHat, or Ubuntu?
This is why we created Pigsty — to develop an battery-included, open-source, local-first PostgreSQL distribution, making it easy for everyone to access and utilize a quality database service. Due to space limits, the detailed story is for another time.
参考阅读
2022-08 《PostgreSQL 到底有多强?》
2022-07 《为什么PostgreSQL是最成功的数据库?》
2022-06 《StackOverflow 2022数据库年度调查》
2021-05 《Why PostgreSQL Rocks!》
2021-05 《为什么说PostgreSQL前途无量?》
2018 《PostgreSQL 好处都有啥?》
2023 《更好的开源RDS替代:Pigsty》
2023 《StackOverflow 7年调研数据跟踪》
2022 《PostgreSQL 社区状态调查报告 2022》